下载中心
优秀审稿专家
优秀论文
相关链接
首页 > , Vol. , Issue () : -
摘要
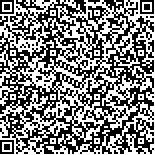
关联是目标感知向目标认知转变中的一个重要步骤,近年来基于计算机视觉技术的目标关联分析已逐渐受到相关领域的研究人员关注,遥感领域关联分析则起步较晚,其中一个重要原因是缺少面向遥感图像的关联数据集。数据集是支撑算法训练、验证以及评估的基础,多样化的数据集能够推动算法持续优化与性能提升。然而,当前尚未有研究对已发布的关联数据集进行全面的归纳和梳理,尤其是在遥感领域,对关联数据集的研究还远不够充分。针对这一问题,本文对相关领域的文献进行全面调研,围绕视觉领域以及遥感领域,对已发布的图像、视频两种模态15个视觉关联数据集以及4个遥感图像关联数据集分别进行了回顾和概述,系统性地介绍了其特点、数据规模、关联类别、三元组数量等内容。同时,本文对已在上述数据集验证的当前主流目标关联分析算法进行了梳理,将现有算法分为基于条件随机场的关联分析算法和基于深度学习的关联分析算法,对每类算法的优缺点进行了分析。最后本文针对当前关联数据集和算法模型的局限性,分别提出了未来可能的研究方向。面向关联数据集,提出一个构建面向时序数据的遥感关联数据集的设想,并初步研制了一套面向遥感图像的关联标注及可视化工具,给未来关联数据集的建设方向提出了优化建议。面向关联算法,本文提出了关联动态分层建模、动态场景图等创新方法,以期为相关领域研究人员提供思路。综上所述,本文将为后续遥感领域关联数据集的构建及相关算法的研究工作提供参考。
Objective Target relationship analysis is to gain a deeper understanding of the target based on the detection task. In recent years, the target relationship analysis based on computer vision has gradually attracted the attention of researchers in related fields, while the relationship analysis algorithm in remote sensing field started late. One of the reasons is the lack of relationship datasets for the remote sensing field. Therefore, this article aims to fill the gap in the review of association datasets, to conduct a detailed review and analysis of relationship datasets and target relationship analysis algorithms in the fields of computer vision and remote sensing, and to provide new ideas for the research and development of target relationship analysis in the remote sensing field. Method In terms of relationship datasets, this article reviews and outlines 15 published image and video visual association datasets and 4 remote sensing image association datasets in the fields of computer vision and remote sensing, and systematically introduces their characteristics, data scale, association categories, number of triples, etc., and summarizes the limitations of existing remote sensing relationship datasets such as inconsistent annotation formats and obvious long-tailed small groups. In terms of target relationship analysis algorithms, this article divides the existing algorithms into relationship analysis algorithms based on conditional random fields and relationship analysis algorithms based on deep learning, and summarizes the advantages and disadvantages of each type of algorithm, and compares the performance of the most advanced algorithms based on the evaluation metric commonly used in target relationship analysis tasks. Finally, the factors that improve the performance of current remote sensing target relationship analysis algorithms are summarized, such as complex semantic relationships, dynamic time-varying scenes, etc. Result In view of the limitations of current relationship datasets and target relationship analysis algorithms, this article proposes possible future research directions respectively. Regarding association datasets, this article proposes a concept of building a remote sensing relationship datasets for time series data and a annotation specifications for remote sensing target relationships, and preliminarily develops a set of association annotation tools for remote sensing images, which provides optimization suggestions for the construction direction of future relationship datasets. Regarding target relationship analysis algorithms, this article proposes innovative methods such as association dynamic hierarchical modeling and dynamic scene graphs, in order to provide ideas for researchers in related fields. Conclusion Target relationship analysis is an important step to move from target perception to cognition. However, the research on target relationship analysis in the field of remote sensing has just started, and there is a lack of discussion of remote sensing target relationship datasets. To address this problem, this article systematically sorts out the existing relationship datasets in the current computer vision and remote sensing fields, and summarizes the limitations of remote sensing relationship datasets. At the same time, this article summarizes the research status of target association algorithms, and demonstrates advantages and disadvantages of existing algorithms according to the algorithm type. Finally, this article proposes a series of possible research ideas for the development of target relationship analysis in the field of remote sensing. In terms of sample accumulation, this article proposes the idea of constructing a remote sensing time series relationship dataset, and in order to unify the annotation format, a set of sample annotation and visualization tools is developed. In terms of algorithm innovation, this article proposes the idea of a dynamic hierarchical relationship reasoning method, which provides new ideas for the future development of target relationship analysis algorithms.