下载中心
优秀审稿专家
优秀论文
相关链接
首页 > , Vol. , Issue () : -
摘要
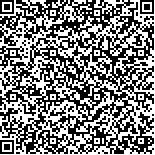
海表温度(Sea Surface Temperature,SST)是研究海洋动力学、海洋-大气相互作用和气候变化的重要指标之一,影响海洋生态系统的平衡和演变。传统的SST获取方式虽然精确,但受限于采样点的数量和覆盖范围,难以满足大尺度、高分辨率的海洋研究需求,而卫星遥感数据可覆盖全球海域,更新频率高,在海洋研究中应用广泛。但是卫星遥感数据在采集过程中,受到天气条件、卫星扫描轨道范围和卫星传感器运行故障等因素的影响,导致SST数据常常存在缺失,一定程度上限制了数据的使用。因此,对遥感数据进行重构以提高其利用效率,获得高质量全覆盖的数据集,对海洋研究及业务化应用具有重要意义。本研究通过引入Inception模块嵌入深度卷积自编码器(DINCAE),提出I-DINCAE模型用于FY-3C卫星南海海表温度产品的数据重构。同时结合研究区多年的实测数据,运用DNN模型对新模型重构的温度数据进行校正。采用11993个独立实测数据点的数据集进行检验,结果表明重构SST与实测SST的RMSE为1.27℃,MAE为0.96℃,R2为0.84,而经过DNN模型校正后,RMSE降至0.57℃,MAE降至0.43℃,R2提高至0.92。基于校正后的海表温度数据,从时间和空间两个维度分析了南海在月度和季度尺度上海表温度的时空分布和变化特征。结果表明:在季节尺度上,南海的SST变化特征明显,表现为夏季SST达到最高值,冬季SST降至最低;在月尺度上,南海SST的变化呈现出正(余)弦波动形式,SST通常在6月份达到极大值,在1月份达到极小值。本研究不仅揭示了南海海洋环境的独特性,也为理解南海海洋生态系统和气候变化提供了重要依据。
The Sea Surface Temperature (SST) is an important indicator for studying ocean dynamics, ocean-atmosphere interaction and climate change, which is closely related to multiple marine environmental factors such as ocean currents, salinity, and nutrient distribution, collectively affecting the balance and evolution of marine ecosystems. Although the traditional SST acquisition methods are precise, they are limited by the number and coverage of sampling points, making it difficult to meet the requirements of large-scale and high-resolution ocean research. Satellite remote sensing data can cover global waters with high update frequency and is widely used in ocean research. However, during the collection process of satellite remote sensing data, SST data is often missing due to factors such as weather conditions, satellite scanning orbit range, and satellite sensor operation failures, which limits the use of data to some extent. Therefore, precise reconstruction of missing data in satellite remote sensing SST data to obtain high-quality and fully covered sea surface temperature datasets is of great significance for ocean research.This study incorporates the Inception module into a deep convolutional autoencoder (DINCAE) and proposes the I-DINCAE model used for data reconstruction of sea surface temperature products with FY-3C satellite in South China Sea. An improved data interpolation convolutional autoencoder (I-DINCAE) is used to reconstruct the missing SST data in the South China Sea from 2014 to 2020, and the reconstruction accuracy of the DINCAE model and I-DINCAE model is compared and analyzed. In order to further improve the accuracy of SST data, deep neural networks (DNNs) were used to calibrate satellite data in combination with measured data, thereby optimizing the quality of the sea surface temperature dataset. Finally, based on the corrected sea surface temperature data, spatio-temporal variation analysis is conducted to reveal the characteristics of sea surface temperature changes. At the same time, combined with many years of measured data, DNN model is used to calibrate the reconstructed temperature data of the new model. A dataset of 11993 independent measured data points was used for testing, the results show that RMSE, MAE and R2 of reconstructed SST and measured SST were 1.27℃, 0.96℃ and 0.84, and after DNN model correction, RMSE decreased to 0.57℃, MAE decreased to 0.43℃ and R2 increased to 0.92. Based on the corrected SST data, the spatio-temporal distribution and variation characteristics of SST in the South China Sea at monthly and quarterly scales are analyzed from two dimensions of time and space. The results show that: on the seasonal scale, the SST of the South China Sea has obvious variation characteristics, which shows that the SST reaches the highest value in summer and the SST decreases to the lowest value in winter. On the monthly scale, the variation of SST in the South China Sea presents sine(cosine) wave form, with SST usually reaching a maximum value in June and a minimum value in January. This study not only reveals the uniqueness of the marine environment in the South China Sea, but also provides an important basis for understanding the marine ecosystem and climate change in the South China Sea.