下载中心
优秀审稿专家
优秀论文
相关链接
首页 > , Vol. , Issue () : -
摘要
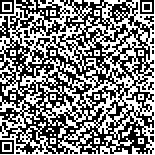
高时空分辨率归一化植被指数(Normalized Difference Vegetation Index,NDVI)数据于作物生长监测及参数反演等具有不可或缺的重要性。卫星遥感数据的红波段和近红外波段可作为NDVI的重要数据源。然而,由于卫星传感器功率的限制,所获取遥感数据的空间分辨率和时间分辨率之间通常存在相互制约,难以获得兼具高时空分辨率的NDVI数据。时空融合技术旨在融合具有高时间分辨率、但空间分辨率较低的NDVI数据和具有高空间分辨率、但时间分辨率较低的NDVI数据,以生成兼具高时空分辨率NDVI数据。然而,NDVI时空融合面临一较大挑战,即已知辅助数据所在时刻与预测时刻之间,地表覆盖通常呈现较大变化。为应对这一挑战,本文提出了一种耦合空域重建的时空融合方法(Spatio-temporal fusion then spatial reconstruction,STFSR)方法,用于NDVI的时空融合。STFSR方法充分利用了在时间上接近预测时刻,但含数据缺失(遥感影像云覆盖导致)的高空间分辨率数据来辅助NDVI时空融合预测。该类辅助影像的利用,有效减小了NDVI变化对时空融合的影响。在本文所选取的三个实验区中,所提出的STFSR方法被证明比常用的时空自适应反射率融合模型(Spatial and Temporal Adaptive Reflectance Fusion Model,STARFM)和基于空间加权和虚拟图像对的时空融合(spatial weighting-based virtual image pair-based spatio-temporal fusion,VIPSTF-SW)方法,具有更高的精度。三个实验区中STFSR方法的平均均方根误差(Root Mean Square Error,RMSE)比STARFM和VIPSTF-SW分别降低了0.0217和0.0188,平均相关系数(Correlation Coefficient,CC)分别提升了0.0820和0.0742,相对全局综合误差(Relative Global-dimensional Synthesis Error,ERGAS)分别降低了4.3170和3.8535。此外,当辅助数据云区域面积增大时,STFSR方法虽在精度上呈现下降态势,但整体上仍优于STARFM和VIPSTF-SW方法。STFSR方法为高时空分辨率NDVI数据的生成提供了一种新的思路,且鉴于其原理与优势,该模型于其他高时空分辨率植被指数数据,例如增强型植被指数(EVI)和叶面积指数(LAI)的生成亦具有可观的应用潜力。
Normalized Difference Vegetation Index (NDVI) images with fine spatial and temporal resolutions are important data for real-time precise vegetation monitoring. Remote sensing image, as an important data source for producing NDVI data, however, always present a trade-off between the spatial and temporal resolutions due to the limitation in the power of satellites. Generally, sensors with fine spatial resolution always have a long revisit time (e.g., Landsat images), while sensors with a short revisit period always have coarse spatial resolution (e.g., Moderate-resolution Imaging Spectroradiometer (MODIS) images). Spatio-temporal fusion technique can be applied to generate NDVI images with both fine spatial and temporal resolutions, by fusing NDVI images acquired from these two categories of sensors. The existing spatio-temporal fusion methods, however, suffer from a long-standing challenge, that is, the NDVI change between the images at the known and prediction times, which restricts the accuracy of spatio-temporal fusion prediction greatly. In this paper, a spatio-temporal fusion then spatial reconstruction (STFSR) method was proposed to cope with the NDVI change issue in predicting the 30 m Landsat NDVI images. Generally, when predicting the missing Landsat NDVI image by spatio-temporal fusion, a pair of spatially complete fine and coarse spatial resolution NDVI images is also required (probably temporally far from the prediction time). Except for the original auxiliary images, the proposed STFSR method also included the fine spatial resolution image temporally closer to the prediction time, but with different degrees of data loss caused by cloud cover (hereafter, simplified as auxiliary cloudy NDVI image) in prediction. The implementation of STFSR is divided into two steps: (1) Reconstructing the non-cloud area (the corresponding non-cloud area in the auxiliary cloudy image, but in the image to be predicted) using the spatial and temporal adaptive reflectance fusion model (STARFM). (2) Reconstructing the cloud area (the corresponding cloud area in the auxiliary cloudy image, but in the image to be predicted) by a spatial-temporal random forest (STRF) algorithm, a spatial reconstruction method integrating the information from both fine and coarse spatial resolution NDVI images. In the experiments in three regions, the effectiveness of the proposed STFSR method was evaluated, by comparing with two commonly used spatio-temporal fusion methods, the STARFM and the spatial weighting-based virtual image pair-based spatio-temporal fusion (VIPSTF-SW) algorithms. The results demonstrate that the proposed STFSR can produce greater accuracy than the other two methods for all three regions. Furthermore, when the cloud coverage increases to a certain percentage (e.g., 80%) in the auxiliary cloudy image, the STFSR method can still provide a more satisfactory prediction than two benchmark methods. Specifically, the average Root Mean Square Error (RMSE) of STFSR is 0.0217 and 0.0188 smaller than that of STARFM and VIPSTF-SW, respectively. The corresponding average Correlation Coefficient (CC) is 0.0820 and 0.0742 larger, and the corresponding average Relative Global-dimensional Synthesis Error (ERGAS) is 4.3170 and 3.8535 smaller. The proposed STFSR method takes full advantage of the important information in the cloudy, but temporally closer NDVI image, which fails to be utilized in existing spatio-temporal fusion methods. Generally, the proposed STFSR method provides a flexible solution to deal with the NDVI change in spatio-temporal fusion. Moreover, this model has great potential for the generation of other vegetation index data with fine spatial and temporal resolutions, such as the Enhanced Vegetation Index (EVI) and the Leaf Area Index (LAI).