下载中心
优秀审稿专家
优秀论文
相关链接
首页 > , Vol. , Issue () : -
摘要
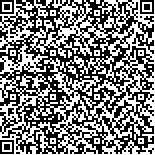
森林土壤有机碳(Soil Organic Carbon,SOC)是反映森林土壤质量的重要标准,很大程度上影响森林树木的生长,对林业的可持续发展起着十分重要的作用。探索应用高光谱影像反演天然次生林土壤有机碳含量的可能性至关重要,可以为长时间序列、大尺度的森林土壤有机碳估测提供技术支持。本研究以天然次生林SOC为研究对象,在东北林业大学帽儿山实验林场随机抽取67个样点,分别采集0-5cm、5-15cm、15-30cm土壤并实测SOC含量,并取三层均值作为0-30cm SOC含量。基于资源1F高光谱影像,计算光谱曲线的一阶微分、二阶微分、倒数对数、植被指数特征,并结合数字高程模型(Digital Elevation Model, DEM)、土壤水分和森林地上生物量(Aboveground biomass, AGB)数据集,使用递归特征消除法进行特征筛选。应用随机森林(Random Forest, RF)、极端梯度提升(extreme Gradient Boosting, XGBoost)、支持向量机回归(Support Vector Regression, SVR)、最小二乘回归(Ordinary Least Squares Regression, OLS)四种模型,分别对SOC进行估测,并选择最佳模型反演帽儿山实验林场不同深度SOC含量。结果显示:在土壤分层反演模型中均为XGBoost估测精度最高,0-30cm、0-5cm、5-15cm、15-30cm四层模型R2分别为0.54、0.54、0.46和0.30,RMSE分别为21.28g/kg、44.25g/kg、15.72g/kg和12.56g/kg。反演得到的帽儿山林场天然次生林0-30cm、0-5cm、5-15cm和15-30cm、四层的SOC平均值分别为67.20g/kg、88.87g/kg、46.92g/kg、40.12g/kg,森林SOC含量随土壤深度增加而减小。不同林分类型的SOC含量存在差异,SOC含量排序从大到小为阔叶林、针阔混交林、针叶林。高光谱影像所包含的波段信息为模型的建立提供了可能,但是过多的波段数量造成了数据冗余,导致模型估测精度的降低,采用递归特征消除法可以筛选出最佳特征组合,有效降低特征数量,提升模型估测精度。710-850nm波段的微分特征对天然次生林SOC的反演较为有效,地形因子对于15cm以上的SOC影响较大,土壤水分和地上生物量对于5-15cm层SOC影响较强。应用高光谱影像结合DEM、土壤水分及地上生物量数据可以有效地反演天然次生林SOC,为基于多期高光谱影像的长时间序列、大尺度的天然次生林SOC反演提供技术支持。
Forest soil organic carbon (SOC) is a critical indicator of forest soil quality, significantly affecting the growth of forest trees and playing an essential role in the sustainable development of forestry. It is imperative to investigate the potential of utilizing hyperspectral images to accurately determine the SOC in natural secondary forests. This would aid in providing technical assistance for estimating forest soil organic carbon on a long-term and large-scale basis. With a focus on the SOC of natural secondary forests, this study randomly selected a total of 67 samples in the Maoershan Experimental Forest Farm of Northeast Forestry University. Soil samples were collected from three different depths: 0-5cm, 5-15cm, and 15-30cm. The SOC content was measured in each sample, and the mean of the three layers was calculated as the SOC content for the 0-30cm depth. The hyperspectral image of ZY1F was analyzed to calculate the first-order differential, second-order differential, reciprocal logarithm of the spectral curve, and vegetation indices. The recursive feature elimination (RFE) method was then employed to screen the features, taking into account the digital elevation model (DEM), soil moisture, and forest aboveground biomass (AGB) datasets. Three machine learning models, namely random forest (RF), extreme gradient boosting (XGBoost), support vector regression (SVR), and ordinary least squares regression (OLS) were employed to estimate the SOC content; and the best model was chosen to estimate the SOC at various depths. The results showed that XGBoost had the highest accuracy in various soil depths: the R2 of the soil depth of 0-30cm, 0-5cm, 5-15cm, and 15-30cm are 0.54, 0.54, 0.46, and 0.30, respectively, and the RMSE are 21.28g/kg, 44.25g/kg, 15.72g/kg, and 12.56g/kg, respectively. The average SOC values in the natural secondary forest of Maoershan Forest Farm were estimated to be 67.20g/kg, 88.87g/kg, 46.92g/kg, and 40.12g/kg for the 0-30cm, 0-5cm, 5-15cm, and 15-30cm soil layers, respectively. The SOC concentration in the forest declined as the soil depth increased. Variations in SOC content exist across various forest types, and the SOC is ordered in descending order as follows: broad-leaved forest, mixed coniferous and broad-leaved forest, and coniferous forest. The band information from hyperspectral images enables the estimation of the SOC in forests. However, the large number of bands leads to data redundancy, which in turn reduces the accuracy of the model"s estimates. The RFE method can be employed to identify the optimal combination of features, thereby reducing the amount of features and enhancing the accuracy of model estimate. The differential characteristics of the 710-850nm bands in hyperspectral images are extremely beneficial for accurately estimating the SOC in natural secondary forests. Topographic factors exert a more significant influence on the SOC at depths above 15 cm, while soil moisture and AGB have a more pronounced effect on SOC in the 5-15 cm layer compared to other factors. The integration of hyperspectral images with DEM, soil moisture, and AGB data can accurately estimate the SOC content of natural secondary forests. This approach offers valuable support for estimating long-term and large-scale SOC of natural secondary forest based on multi-period hyperspectral images.