下载中心
优秀审稿专家
优秀论文
相关链接
首页 > , Vol. , Issue () : -
摘要
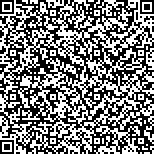
语义分割在遥感场景理解中起着至关重要的作用,其目的是为遥感图像中的每个像素分配类标签。然而遥感图像拥有复杂的前景和背景信息,已有的语义分割网络,如双模态分割方法,分别提取图像特征,故存在特征融合困难与特征过于单一等问题。因此,本文提出基于三分支集成网络的高分辨遥感图像语义分割算法。首先为语义特征和空间特征分别设计了不同的特征提取分支,以充分利用图像的语义和空间信息。其次,提出一致性分支的概念,旨在学习语义和空间一致性的特征,防止在特征融合过程中出现特征过于单一的问题,从而提高网络的分割性能。最后,提出多尺度特征融合模块对三分支特征进行加权融合。此外,由于遥感图像不同的增强视图表示完全不同的对象,本文提出一种关注空间一致性的随机采样裁剪数据增强方法,并加入了混合损失函数,解决数据集中类别数量不平衡问题,有效缓解因遥感数据集数据量过小而导致的过拟合问题。实验结果表明,本文所提算法在ISPRS Potsdam和Vaihingen数据集上的平均交并比达到87.84%和87.49%,证明本算法能够高效地提取并融合高分辨率遥感图像的语义和空间特征,从而提高遥感图像分割的准确率。
Remote sensing images are geospatial observation data with wide coverage, diverse spectral information, and variable target structures, typically collected by platforms such as remote sensing satellites, drones, or airships. With multiple spectral bands like visible light and infrared, remote sensing images provide rich information for representing surface features. As the coverage of remote sensing images expands and the variety of objects increases, the difficulty of extracting information from images also increases. Early methods for interpreting remote sensing images cannot meet the current demands for remote sensing image interpretation. Deep learning exhibits strong capabilities in extracting both shallow and high-level semantic information, which is of practical significance and research value in semantic segmentation of remote sensing images. Existing semantic segmentation networks, such as multimodal segmentation methods, often face challenges in feature fusion and overly simplistic features due to separate feature extraction.