下载中心
优秀审稿专家
优秀论文
相关链接
首页 > , Vol. , Issue () : -
摘要
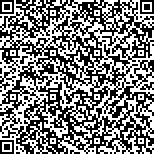
精确的单木树种信息对于森林资源监测、经营管理、生态系统评估和生物多样性研究至关重要。基于机载高光谱和激光雷达(Light Detection and Ranging, LiDAR)数据的结合,为森林树种分类带来了新的机遇。虽然近年来已有许多基于高光谱和LiDAR数据的小范围树种分类研究,但在实际更大范围的森林场景中,由于树种类型多样和林龄结构复杂,对影响机载高光谱与LiDAR数据分类精度的因素及其定量研究仍然不足。本文以塞罕坝机械林场为例,设计了四种分类策略,探讨了机载多航带影像光谱一致性校正处理、树高特征引入以及单木冠层信息对人工林树种精细分类精度的影响。这四种策略分别为:①未经过二向性反射率分布函数(Bidirectional Reflectance Distribution Function,BRDF)校正的多航带影像衍生的植被指数,②经过BRDF校正后的多航带影像衍生的植被指数,③BRDF校正影像衍生的植被指数+树高特征,④BRDF校正影像衍生的植被指数+树高特征+单木冠层信息。研究表明,(1)基于单木分割信息的树种分类可有效地减少同一树冠内多像元对应多树种的分类错误,对树种分类精度提升贡献十分显著(10.74%); (2)通过对机载影像进行辐射一致性校正,虽然降低了多航带影像间由于太阳-观测几何造成的同一树种光谱反射的差异,但对树种分类精度的提升有限(3.48%);(3)在不同树种具有相似的垂直结构,或者同一树种分布在多个林龄阶段的地区,冠层高度(Canopy Height Model, CHM)对树种分类精度的提升贡献几乎可忽略(0.67%)。综上所述,虽然结合机载高光谱和LiDAR数据的树种精细分类在较大范围人工林中表现出良好的应用价值和潜力,但仍需对众多影响因素进行深入分析与优化,以便为更有效地开展森林资源高效监测、管理和其他林业遥感应用奠定科学基础。
Objective This research aims to examine the key factors influencing the accuracy of tree species classification using airborne hyperspectral data combined with Light Detection and Ranging (LiDAR) in forest environments. Accurate identification of individual tree species is essential for effective forest resource monitoring, management, ecosystem assessment, and biodiversity conservation. While many small-scale studies have explored tree species classification in forests with diverse species compositions and complex age structures, achieving this over larger areas remains a significant challenge. This study focuses on evaluating the effects of spectral consistency correction, canopy height information, and individual tree canopy segmentation on classification accuracy. Saihanba Mechanical Forest Farm, a large-scale artificial plantation, was selected as the study site to explore these factors. Method To assess the impact of different factors on tree species classification accuracy, the research utilized a Random Forest classification algorithm and developed four distinct classification strategies. The first strategy used vegetation indices derived from multi-flightline images without applying Bidirectional Reflectance Distribution Function (BRDF) correction. The second strategy incorporated BRDF correction into the multi-flightline images before deriving vegetation indices. The third approach integrated canopy height information, specifically the Canopy Height Model (CHM), with the BRDF-corrected vegetation indices. The fourth and final strategy combined BRDF-corrected vegetation indices, CHM, and individual tree canopy segmentation data. The classification accuracy of each strategy was systematically compared to quantify the contribution of each factor toward improving tree species classification precision. Result The results indicated that individual tree canopy segmentation significantly reduced misclassification errors arising from the mixing of multiple species within a single canopy, leading to a notable 10.74% improvement in classification accuracy. Using the Random Forest model’s feature importance ranking, individual tree segmentation emerged as the most critical factor, followed by BRDF correction, and then the canopy height model. Although BRDF correction reduced spectral reflectance variability caused by differing sun-observation geometries across flight strips, it only led to a modest improvement in classification accuracy of 3.48%. The introduction of the Canopy Height Model (CHM) yielded minimal gains in accuracy, contributing just 0.67%, particularly in areas with uniform vertical forest structures or species spanning multiple age cohorts. Conclusion This study demonstrates that integrating airborne hyperspectral data with LiDAR holds substantial promise for enhancing tree species classification in large-scale artificial plantations. Among the factors analyzed, individual tree segmentation proved to be the most impactful in improving accuracy. In contrast, the relatively minor influence of BRDF correction and canopy height features underscores the need for further refinement and optimization. Overall, the findings emphasize the importance of considering multiple factors in remote sensing workflows to enhance the efficiency and accuracy of forest resource monitoring, management, and other forestry-related applications, especially in expansive forest environments. These insights provide a valuable theoretical foundation and practical recommendations for future forest management and ecological monitoring efforts.