下载中心
优秀审稿专家
优秀论文
相关链接
首页 > , Vol. , Issue () : -
摘要
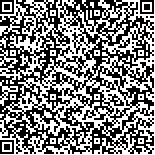
高光谱图像分类旨在为高光谱图像中每一像素赋予所属类别,是遥感领域中的一项重要应用。近年来,对比学习由于其良好的挖掘数据关键特征的能力,被广泛运用于高光谱图像分类任务中。但是目前自监督对比学习范式大多采用两阶段方案训练网络,在预训练阶段难以避免将同一类的物体定义为负样本,往往导致类内差距变大。此外,对比学习算法一般采用裁剪、旋转等数据增强方式生成正样本,生成正样本的多样性较为受限。针对上述问题,本文提出了一种基于多尺度监督对比学习的高光谱图像分类网络。具体而言,本文提出了多尺度对比特征学习网络逐层次提取光谱特征和多尺度空间特征,构建了类级对比策略将来自同一类别的不同尺度样本给予紧密一致的表示,进一步拉近特征空间中正样本之间距离,使类内数据更加聚集。然后,提出了空谱混合概率导向融合分类网络,设计了自适应机制动态调整特征融合的权重,挖掘光谱与空间信息的潜在关联,从而实现精准的分类结果。在Houston 2013、WHU-Hi-LongKou和Pavia University高光谱数据集上的试验结果表明,本文方法与其他主流深度学习方法相比表现出更加优越的分类性能。
Hyperspectral image classification, which aims to assign a belonging category to each pixel in a hyperspectral image, is an important application in the field of remote sensing. In recent years, contrastive learning has been widely used in hyperspectral image classification tasks due to its good ability to mine key features of data. However, most of the current self-supervised contrastive learning paradigms use a two-stage scheme to train the network, and it is difficult to avoid defining objects in the same class as negative samples in the pre-training stage, which often leads to a wider intra-class gap. In addition, contrastive learning algorithms generally use data enhancement methods such as cropping and rotating to generate positive samples, and the diversity of generated positive samples is more limited. In this paper, a hyperspectral image classification network based on multi-scale supervised contrastive learning is proposed to solve the above problems. The method aims to extract multiscale spatial features and spectral features level by level, and adaptively fuse the features to generate the final results. This paper proposes a Multiscale Supervised Contrastive Learning Network (MSCLN) for hyperspectral image classification, which includes two parts: a multiscale contrastive feature learning network and a spatial-spectral hybrid probability-directed fusion classification network. In the multiscale contrast feature learning network, a spectral-guided branch and a spatial feature extraction branch that introduces an attention mechanism are designed to extract spectral-spatial features level by level. Then, two multi-scale spatial features of the same object are constructed as positive samples by introducing label information. Specifically, 2n views can be generated for n objects, in which all views of the same kind of objects are positive samples of each other and the rest are negative samples. Finally, in the spatial-spectral hybrid probability-directed fusion classification network, the learnable parameters are set to integrate the spectral-spatial features to obtain the final classification probability. By co-training the two networks, more accurate classification results can be obtained. In three public hyperspectral datasets, Houston 2013, WHU-Hi-LongKou and Pavia University, 50, 80 and 50 labeled samples were randomly selected from each category as training sets, respectively. The overall classification accuracy of the proposed algorithm reached 96.20%, 99.20% and 98.96%, respectively, and the classification performance was better than that of the other comparison methods. The method extracts the discriminative spectral-spatial features hierarchically by MSCLN, and introduces the labelling information to construct the two multiscale spatial features of the same object as positive samples. It makes the same kind of sample distance more aggregated while pushing away the inter-class distance. Finally adaptive fusion of spectral-spatial features to obtain an excellent classification map.