下载中心
优秀审稿专家
优秀论文
相关链接
首页 > , Vol. , Issue () : -
摘要
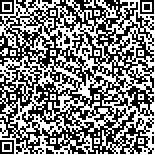
旋转目标检测算法在遥感领域中有着广泛的应用。遥感图像中目标尺度变化大、目标密集分布、相似地物易混淆、背景复杂干扰多以及图像细节不足,现有的旋转目标检测算法通常存在较高的计算负担,并且在精度上仍有提升的空间。针对上述问题,本文改进当前领先的YOLOv9检测器,开发了一种高效而准确的遥感图像旋转目标检测器RSO-YOLO(YOLO for Remote Sensing Images and Oriented Bounding Box)。首先,提出了一种低照度遥感图像辅助数据增强模块,用于改善弱光、噪点、模糊和对比度不足等问题;其次,设计了一个解耦的角度预测头,使算法拥有对遥感目标方向的感知能力;然后,在模型中引入基于卡尔曼滤波的交并比(KFIoU)损失,以解决旋转目标表示引起的角度周期性问题,使用分布焦点损失(DFL)学习旋转边界框的分布,减少高斯建模方法中近正方形目标的角度不准确问题;提出一种面向旋转目标检测的动态标签分配策略,在分配过程中综合考虑了交并比(IOU)与类别得分(Scores),从而构建更好的能够反映目标特性的样本空间;最后使用基于海林格距离的概率交并比(ProbIoU)进行非极大值抑制,减少非极大值抑制的计算负担。在DIOR-R公开数据集上开展实验,与多个典型的旋转目标检测方法进行了比较,本文方法综合检测精度为81.1%mAP,位居第一,且能够保证检测的实时性。使用辅助数据增强模块后可提高1.5%mAP。实验表明RSO-YOLO能够同时兼顾旋转目标检测的速度和准确性,使得模型在实际应用上相较于其他方法有一定的优势。
Rotated object detection is a fundamental task in the field of remote sensing, essential for accurately identifying and localizing objects with arbitrary orientations in aerial or satellite imagery. Despite its importance, existing rotated object detection algorithms face significant challenges that hinder their performance and practical applicability. These challenges include large variations in target scales, dense distributions of targets leading to occlusions, confusion with similar objects due to limited distinguishing features, complex background interference, and a general lack of image details owing to factors like low resolution or adverse imaging conditions. These issues often come with high computational burdens and limitations in detection accuracy. To address the challenges of rotated object detection in remote sensing images, this paper improves upon the state-of-the-art YOLOv9 detector by developing a new high-performance rotated object detector that balances detection accuracy and inference speed. Firstly, an auxiliary data enhancement module for low-light remote sensing images based on the Retinex algorithm is introduced to improve problems related to low light, noise, blurring, and lack of contrast. Secondly, a decoupled angle prediction head is designed to enable the algorithm to perceive the orientation of remote sensing targets. Next, a Kalman filter-based Intersection over Union (KFIoU) loss is incorporated into the model to address the periodicity issue caused by rotating object representation, using Distribution Focal Loss (DFL) to predict angles and solve the angle representation problem for nearly square objects within Gaussian modeling methods. Additionally, a dynamic label assignment strategy for rotated object detection is proposed, which takes into account both IoU and score values during the assignment process and incorporates a distance penalty term, thereby constructing a sample space that better reflects the characteristics of the targets. Finally, during non-maximum suppression, we use probabilistic Intersection over Union and merging rate (ProbIoU) based on the Hellinger distance to remove some redundant candidate boxes, thereby reducing computational burden. We evaluated the proposed RSO-YOLO detector on the publicly available DIOR-R dataset. Experimental results show that our method achieved a mean Average Precision (mAP) of 81.1% on this dataset, surpassing several typical rotated object detection methods and achieving top rank in detection accuracy. Notably, the introduction of the auxiliary data enhancement module contributed to a 1.5% increase in mAP, demonstrating its effectiveness in enhancing detection performance under low-light and poor-quality imaging conditions. Moreover, we verified the model"s generalization ability on the DOTA dataset, where the results were also outstanding. The model maintained real-time detection capabilities, highlighting its practical applicability for time-sensitive remote sensing tasks. In summary, the RSO-YOLO detector demonstrates superior performance compared to existing methods by integrating an auxiliary data enhancement module, utilizing a decoupled angle prediction head, improved loss functions, a dynamic label assignment strategy, and an efficient non-maximum suppression method. Experimental results confirm that RSO-YOLO not only achieves higher accuracy but also operates efficiently, making it particularly advantageous for practical applications in remote sensing where both speed and precision are critical. Future work may involve further optimization of the proposed methods and exploring their application to other types of imagery and detection tasks.