下载中心
优秀审稿专家
优秀论文
相关链接
首页 > , Vol. , Issue () : -
摘要
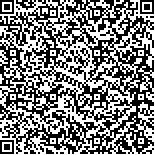
在现阶段星载GNSS反射信号(Global Navigation Satellite System-Reflectometry,GNSS-R)海面高度反演的误差研究中,使用经典误差模型对反演结果修正后仍存在较大误差。针对该问题,提出一种基于神经网络与注意力机制结合训练的误差补偿模型,通过误差补偿模型对海面高度反演结果进行修正,并使用DTU验证模型来评估修正精度。利用风云三号E星的星载GNSS反射信号中的DDM(Delay-Doppler Mapping)数据进行实验,结果显示:GPS(Global Positioning System)反射信号的数据使用误差补偿模型修正后的MAE(Mean Absolute Error)为1.74米,BDS(Beidou Navigation Satellite System)反射信号的数据使用误差补偿模型修正后的MAE为0.97米,相较经典误差模型修正精度提升了约80%;同时,本文模型相较于使用随机森林算法和CNN算法训练的模型精度稍有提升。实验证明,提出的误差补偿模型有效的修正了星载GNSS-R海面高度反演误差。
Objective In the current research on sea surface height inversion from satellite-borne GNSS reflected sig-nals, classical algorithms are usually used to invert sea surface height. However, due to the existence of multiple complex errors such as inaccurate receiver orbit, system error, ionosphere error, troposphere error, etc., the results inverted using classical algorithms are mostly of low accuracy. Therefore, an error model is needed to correct the inversion results. Classic error models generally improve the accuracy of sea surface height inversion by correcting common errors such as tropospheric error, ionosphere error, and antenna baseline attitude error, but there are still large errors that cannot be corrected. To address this problem, this paper proposes an error compensation model based on the combined training of neural networks and atten-tion mechanisms to correct the sea surface height inversion results. Method This paper designs a CNN-AM training method that combines a Convolutional Neural Network (CNN) model with an Attention Mechanism (AM) to accurately train the error of sea surface height inversion from satellite-borne GNSS reflection sig-nals, generate an error compensation model to replace the classical error model, and improve the accuracy of sea surface height inversion. Result The proposed model was compared with the classic error model, CNN model, and random forest model, and tested on about 2 million DDM (Delay-Doppler Mapping) data of the FY-3E dataset. The evaluation indicators used MAE (Mean Absolute Error) and RMSE (Root Mean Square Error). The MAE of the GPS (Global Positioning System) reflected signal data corrected by the error compensation model was 1.74 meters, and the RMSE was 2.25 meters; the MAE of the BDS (Beidou Naviga-tion Satellite System) reflected signal data corrected by the error compensation model was 0.97 meters, and the RMSE was 2.16 meters. Compared with the classic error model, the correction accuracy was improved by about 80%; compared with the random forest model and CNN model, the accuracy was also slightly im-proved. Conclusion This paper proposes an error compensation model based on the training of neural net-work and attention mechanism to correct the sea surface height inversion results. Experiments show that the proposed error compensation model effectively corrects the sea surface height inversion error of space-borne GNSS-R.