下载中心
优秀审稿专家
优秀论文
相关链接
首页 > , Vol. , Issue () : -
摘要
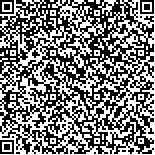
水稻是世界上最重要的粮食作物之一,它提供了全球近50%人口的食物来源。此外,水稻的生长需要大量水资源并且稻田淹水是甲烷排放的主要来源之一。因此,掌握水稻种植的分布区域对粮食安全和生态环境十分重要。为了快速准确绘制水稻分布图,本研究基于NDVI与MNDWI时间序列,构建了新型光学水稻指数(New Optical Paddy Rice Index,NOPRI)。并在4个具有不同气候以及水稻种植模式的区域进行了应用。结果表明,NOPRI能够准确生成水稻分布图,总体精度在0.945以上,F1分数在0.907以上。与现有方法和数据集的相比,NOPRI在地物异质性较大的区域具有更高的适用性。由于NOPRI的构建简单,阈值稳定,因此其具备大尺度的水稻制图能力。
关键词:
水稻 Sentinel-2 时间序列 时序谐波分析 区域性水稻指数Rice is undeniably one of the most crucial food crops globally, serving as the primary source of sustenance for nearly 50% of the world"s population. Its significance extends beyond mere food provision, as the cultivation process demands substantial water resources, and flooded rice fields contribute significantly to methane emissions, thereby presenting formidable challenges to both food security and ecological equilibrium. In this context, precisely determining the distribution areas of rice cultivation emerges as a pivotal task for effective food security and ecological environment management. Traditional manual survey methods have long been constrained by their limited scope and labor-intensive nature. In contrast, remote sensing technology has emerged as a powerful alternative, enabling the efficient monitoring of large-scale and long-term rice cultivation. By capitalizing on imagery data procured from satellites or aerial platforms, remote sensing offers comprehensive coverage, facilitating in-depth and detailed monitoring of rice cultivation regions. To address the need for rapid and accurate rice distribution mapping, this research has introduced an innovative optical paddy rice index known as NOPRI. The construction of NOPRI is a meticulously designed process. Initially, leveraging the Google Earth Engine (GEE) platform, the Sentinel-2 Level 1C image products corresponding to the target area and year were acquired. Subsequently, cloud masking was executed to eliminate the interference of cloudy pixels, followed by the calculation and generation of the time series of the Normalized Difference Vegetation Index (NDVI) and the Modified Normalized Difference Water Index (MNDWI). These indices play a fundamental role in characterizing the growth and environmental conditions of rice. Harmonic analysis was then employed to reconstruct the NDVI and MNDWI time series, effectively enhancing the visualization and understanding of the periodic patterns inherent in rice growth. The coefficients derived from this time series harmonic analysis were exploited to quantitatively assess the unique time series characteristics of rice. Through a concise statistical analysis of a relatively small number of samples, the feature coefficients were extracted, refined, and combined. Notably, the characteristics of MNDWI during the critical rice flooding period were integrated into the index formula, endowing NOPRI with enhanced discriminatory power. This study achieved remarkable success in mapping the rice distribution in Region 1 in 2019, Region 2 in 2019, Region 3 in 2022, and Region 4 in 2018 by applying index threshold segmentation. To rigorously evaluate the accuracy of these maps, a comprehensive assessment was conducted using 7,001 validation sample points. The outcomes revealed that the overall accuracy of NOPRI surpassed 0.945, and the F1 score exceeded 0.907, providing strong evidence of the high reliability of the mapping results. In the evaluation of the mapping effect, a detailed visual comparison between the enlarged views of the rice distribution maps and the Sentinel-2 RGB images demonstrated a striking similarity. When contrasted with the Synthetic Aperture Radar-based Paddy Rice Index (SPRI) and existing rice datasets, NOPRI exhibited superior extraction accuracy and more precise mapping effects. However, it is important to note that the performance of NOPRI is susceptible to the quality of optical data. In research areas where the long-term quality of optical data is suboptimal, the extraction accuracy and mapping effect of the rice index may be compromised. Future research efforts should focus on devising strategies to mitigate the impact of data quality issues and further enhancing the robustness and applicability of NOPRI.