下载中心
优秀审稿专家
优秀论文
相关链接
首页 > , Vol. , Issue () : -
摘要
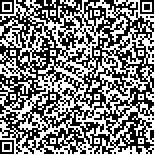
耕地的数量是保证农业可持续发展的基础,快速、精准监测耕地非农化行为对我国粮食生产和安全具有重要意义。为精准监测乱占耕地进行非农建设的行为,本文提出一种准确的高分辨率遥感图像耕地非农化行为分割的新方法。首先,根据多时相遥感数据构建亚米级的潜在非农化区域的建筑物样本数据集;然后,使用本文设计的耕地非农化行为监测深度学习模型(DHRformer)完成对潜在非农化区域内建筑物的提取。DHRformer模型由高分辨率网络及双分支解码结构组成,通过多尺度融合和扩张策略增强非农建筑的特征信息,从而获得更丰富的非农建筑细节信息;最后,选取怀化市鹤城区为研究区域,使用本文提出的DHRformer模型与几种流行的语义分割方法进行对比。实验结果表明,本文方法对潜在非农化建筑的分割和边缘表征具有更好地表现,在F1-score、mAcc及MIoU精度指标分别达到89.81%、89.37%和80.35%,分割精度优于现有方法。因此,本文方法在耕地非农化行为监测任务中具有较高的实用价值。
The amount of arable land is the basis for ensuring the sustainable development of agriculture, and rapid and precise monitoring of the non-farming behavior of arable land is of great significance to China"s food production and security. To accurately monitor the indiscriminate occupation of arable land for non-farming construction, this paper proposes a new method for accurate segmentation of the non-farming behavior of arable land in high-resolution remote sensing images. Firstly, a sub-meter-level sample dataset of buildings in potential non-agriculturalized areas is constructed based on multi-temporal remote sensing data; then, the extraction of buildings in potential non-agriculturalized areas is completed using the Deep Learning Model for Monitoring Non-Agriculturalization Behavior of Cultivated Land (DHRformer) designed in this paper. The DHRformer model consists of a high-resolution network and a two-branch decoding structure, which enhances the feature information of non-agricultural buildings through multi-scale fusion and expansion strategies so as to obtain richer information about the details of non-agricultural buildings. Finally, Hecheng District, Huaihua City, is selected as the study area, and the DHRformer model proposed in this paper is used to compare with several popular semantic segmentation methods. The experimental results show that this paper"s method has better performance in segmentation and edge characterization of potential non-agriculturalized buildings, reaching 89.81%, 89.37%, and 80.35% in F1-score, mAcc and MIoU accuracy metrics, respectively, and the segmentation accuracy is better than that of existing methods. Therefore, the method in this paper has high practical value in the task of monitoring the non-farming behavior of arable land.