下载中心
优秀审稿专家
优秀论文
相关链接
首页 > , Vol. , Issue () : -
摘要
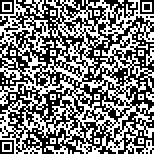
根据地理学第二定律,空间数据及其变量关系的异质性或非平稳性特征逐渐成为空间计量分析的重要内容之一。结合第一定律所阐释的空间依赖性原理,涌现了以地理加权回归分析技术为代表的一系列地理加权建模技术,功能层面覆盖描述性、探索性、解释性和预测模拟等不同分析需求层次。本文系统梳理了地理加权建模技术理论与技术框架,归纳了其共性特点与核心准则,从前提假设、距离度量、权重计算和带宽优选四个方面阐述了地理加权建模技术的基础构成,并从四个分析需求层次讨论了不同地理加权模型的潜在适用范围。但是,现有地理加权建模技术在理论基础、完备性、互补性和时空拓展方面仍然存在一定问题,距离成为一个完整的空间异质性量化分析框架仍然任重而道远。
According to the second law of geography, spatial heterogeneity or non-stationarity in spatial data and relationships has increasingly drawn more and more attentions in spatial statistics. To explore this fundamental phenomenon, place or location-specific methods and local statistical techniques that assume data relationships to be spatially variant have been extensively developed. In line with the principle of spatial dependence depicted by the first law of geography, the GW regression technique was first proposed to incorporate spatial weights into location-wise regression model calibrations to highlight spatial heterogeneities in data relationships by outputting spatially varying coefficient estimates. With this distance-decaying schema for calculating spatial weights, a series of geographically weighted (GW) models emerge to conduct fine-scaled spatial analysis in terms of descriptive, explanatory, interpretive and predictive scenarios, including GW descriptive statistics, a number of basic GW regression and extensions, GW discriminant analysis, GW principal component analysis, GW machine learning, GW artificial neural network. These GW models form a continually evolving technical framework for identifying spatially non-stationary features or patterns in a variety of disciplines or fields, including geography, social science, biology, public health, and environment science. In this study, we tried to systematically sort out the theoretical and technical framework of GW models. First of all, we summarized the essence and rules for applying the family of GW models, i.e. catering for spatially heterogeneous or non-stationary features and relationships in geographic variables, outputting location-dependent metrics or estimates via calculating spatial weight matrix the distance-decaying principle of spatial dependence presented by Tobler"s First Law of Geography. As common and fundamental parts of the GW models, we introduce the hypothesis tests of spatial heterogeneity or non-stationarity, general definition of distance metrics in geography, calculation of spatial weights and bandwidth optimization. Regarding descriptive, explanatory, interpretive, and predictive scenarios, the potential usages of each individual GW model are also discussed from four analysis levels. We recommend univariate GW descriptive statistics, e.g., GW average, GW quantile, GW standard deviation, and GW Skewness, to facilitate users" grasping the spatially heterogeneous distribution of a geographic variable. For exploratory data analysis with multivariate spatial data, GW correlation coefficient and GW principal component analysis could be preferable. GW regression and its rich extensions, specifically multiscale GW regression, provide powerful tools in interpretive analysis and have been widely applied. With data relationships studied comprehensively, accurate predictions usually appear as an ultimate target in data analytics. The usages of GW regression and geographically and temporally weighted regression are straightforward for predictions, and the prediction accuracy is further improved when the artificial intelligence technologies are incorporated, e.g. GW machine learning, GW artificial neural network and geographically neural network weighted regression. The increasing popularity of GW models has resulted in the development of several software packages, standalone programs and toolkits, including the R package GWmodel and GWmodelS, a new, free, user-friendly and high-performance standalone software that incorporates spatial data management and mapping tools as well as the GW model functions. However, there is still a long way to go before GW models being an all-around quantitative analytical framework for spatial heterogeneity due to drawbacks in theoretical foundation, technical completeness and complementarity, and their evolutions to spatio-temporal dimensions.