下载中心
优秀审稿专家
优秀论文
相关链接
首页 > , Vol. , Issue () : -
摘要
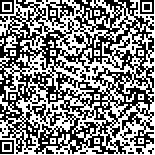
针对不同时相的非同源遥感影像存在的空间异质性问题,本文对全变网络模型(Fully Transformer Network,FTN)进行改进,提出一种端到端的、基于滑窗式特征增强和卷积注意力混合机制的倒塌建筑物变化检测网络模型(Sliding-Window-Shift Attention Convolution mix Network, SWSACNet)。SWSACNet基于FTN的模型框架,使用ACmix(Attention Convolution mix)高效识别非同源影像对中的倒塌建筑物特征,并通过滑窗相似度特征匹配减弱非同源影像中位置偏差的影响。以2023年2月6日土耳其Mw7.8级地震为例,通过获取震前高分二号、Google影像和震后北京三号影像构建倒塌建筑物变化检测数据集,对SWSACNet、FTN等五种变化检测模型进行训练和震区倒塌建筑物提取测试。实验结果表明,SWSACNet识别精度F1 score达80.8%,mIoU为67.8%,均优于其他四类模型。SWSACNet在应用于Fevaipasa、Nurdagi和Islahiye三个测试场景中,模型平均识别精度F1 score为60.84%,表明模型在泛化性能上有待提升。
Change detection networks based on deep learning are widely used in water monitor, urban change, etc.. However, collapsed buildings, as one of the change objectives, are rarely targeted for change detection networks. This study proposes an end-to-end collapsed building extraction model based on a change detection network including the sliding-window feature enhancement and convolution attention mix mechanism, which called SWSACNet (Sliding-Window-Shift Attention Convolution mix Network). SWSACNet is an improvement of Fully Transformer Network (FTN). FTN is a network completely composed of Swin Transformer. Besides, it has a unique frame, which involves four processes: SFE (Siamese Feature Extraction), DFE (Deep Feature Enhancement), PCP (Progressive Change Prediction), DS (Deep Supervision). By encoding and decoding the feature of change objects deeply, FTN is able to learn what the collapsed buildings has changed in two temporal images and suppress irrelevant information, such as emergency tents. ACmix, a blend of convolution and attention mechanism, has been proved better performance than Swin Transformer in mainstream datasets. However, due to the different sensors, platforms, etc., the spatial heterogeneity of target features in different source remote sensing images will affect the accuracy of change detection. Concerning this problem, we designed a similarity sliding window to match the feature maps of two temporal images. Hence, we replace Swin Transformer with ACmix to extract and restore earthquake-damaged features efficiently in the phase of SFE and PCP, and using similarity sliding window to reduce misidentifications of collapsed buildings in different source image pairs before the phase of DFE. Taking the earthquake with 7.8 magnitude on February 6th, 2023, in Turkey as an example, establish a building seismic damage change detection dataset which consists of pre-earthquake Gaofen-2, Google images and post-earthquake Beijing-3 images, and collapsed buildings were extracted based on the SWSACNet, FTN, STANet based on the Siamese self-attention mechanism, DASNet based on a dual-attention fully-convolutional neural network, and the conventional fully-convolutional early fusion FC-EF network. The experimental results show that SWSACNet achieves the highest accuracy with F1 score of 80.8% and mIoU of 67.8%. The ablation experiments of the improved model indicates that SWSACNet obtains highest precision among three structure combinations. Beyond that, by smoothing the BJ-3 image that has higher spatial resolution to make the gradient change rate of image pairs closer, we acquire a new dataset and use it to retrain the five models. We found that the precision of five retrained models increases 1% at least, which also illustrates that appropriately narrowing the gap of gradient change rate of image pairs is an effective preprocessing for models to recognize the collapsed buildings. Finally, applying SWSACNet to Fevaipasa, Nurdagi and Islahiye, the results show that it achieves 60.84% of average F1 score in three scenarios. The application of SWSACNet in three different scenarios presents that the model needs to improve its generalization.