下载中心
优秀审稿专家
优秀论文
相关链接
首页 > , Vol. , Issue () : -
摘要
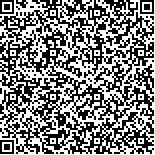
多光谱遥感图像具有能够反映丰富地物特征的光谱信息,但其空间分辨率较低,纹理信息相对不足。相反地,全色遥感图像的空间分辨率高,纹理信息丰富,但缺乏能够反映地物特征的丰富的光谱信息。通过图像融合技术可以将二者进行集成,以达到各自的优势互补,从而使得融合所得的图像能够更好地满足下游任务的需要。为此,本文提出了一种无监督的基于双支路生成对抗网络与Transformer的多光谱与全色遥感图像融合方法。具体地,首先采用引导滤波将源图像(源多光谱和全色遥感图像)分解为呈现图像主体信息的平滑分量与体现图像纹理、细节信息的细节分量;然后,将分解得到的多光谱和全色遥感图像的平滑分量进行级联,将二者分解得到的细节分量也进行级联;其次,将级联后的平滑分量和细节分量分别输入至双支路生成器的平滑支路和细节支路中;接着,针对平滑分量与细节分量各自不同的特性,分别采用Transformer网络和卷积神经网络进行特征信息提取,以便从平滑层分支和细节层分支中分别提取得到全局光谱信息和局部纹理信息;最后,通过生成器和双判别器(平滑层判别器和细节层判别器)之间不断地对抗训练,得到同时具有丰富光谱信息与高空间分辨率的融合图像。通过在公开的数据集上与多个有代表性的方法进行定性与定量的对比实验表明,本文所提方法具有一定优越性,即在主观视觉效果和客观评价指标上均取得了较好的融合效果。
Multispectral remote sensing image has rich spectral information that can reflect ground features, but its spatial resolution is low and its texture information is relatively insufficient. By contrast, panchromatic remote sensing image has high spatial resolution and rich texture information, but lacks rich spectral information that can reflect ground features. In practice, two kinds of images can be integrated into a single one to obtain the complementary advantages from the different images, thereby the fused image can better meet the needs of downstream tasks. To this end, this paper proposes an unsupervised method for fusing the panchromatic and multispectral images based on dual-branch generative adversarial network and Transformer. Specifically, the source images (source panchromatic and multispectral images) are firstly decomposed into smooth and detail components using guided filtering, where the smooth component mainly focuses on the main body of the source image, and the detail component mainly reprents the texture and detail information of the source image; Next, concatenates the decomposed smooth components of the panchromatic and multispectral images, and also concatenates the decomposed detail components of the two kinds of source images; Then, respectively inputs the concatenated smooth and detail components into the smooth and detail branches of the dual-branch generator; Next, according to the different characteristics of the smooth and detail components, respectively utilizes the transformer and CNN to extract the global spectral information from the smooth branch and the local texture information from the detail branch; Then, continuously trains the model in an adversarial fashion between the generator and the dual discriminators (smooth layer discriminator and detail layer discriminator), and finally obtains the fused image with rich spectral information and high spatial resolution. Extensive experiments on the public dataset demonstrate that the proposed method outperforms the state-of-the-art methods both in qualitatively visual effects and in quantitatively evaluated metrics.