下载中心
优秀审稿专家
优秀论文
相关链接
首页 > , Vol. , Issue () : -
摘要
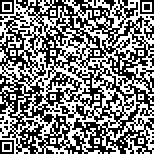
准确估测森林碳储量对于森林生态系统的保护和可持续发展有着重要意义,但是遥感时间序列数据存在大量的噪声,为了提高碳储量的估测精度,开发一种滤波算法,以减少高海拔地区Landsat时间序列数据噪声的干扰。基于1987、1992、1997、2002、2007、2012、2017年国家森林资源连续清查固定样地的数据以及1987—2017年的Landsat时间序列影像。本研究利用Python开发了自适应地形卷积ATC(Adaptive Topography Convolution)算法,该算法考虑到地形因素对于图像质量的影响,在尽可能保留图像细节的情况下去除图像的噪声,并使用Savitzky-Golay滤波、中值滤波对Landsat时间序列数据进行滤波。应用随机森林回归算法RFR(Random Forest Regression)构建香格里拉市高山松碳储量估测模型,选择最优估测模型对1987、1992、1997、2002、2007、2012、2017年高山松碳储量进行反演制图。结果表明:1)从图像的质量评价指标平均绝对误差MAE(Mean Absolute Error)来看,经过ATC算法滤波后的图像质量最好;2)在使用RFR方法的情况下,滤波后的数据均表现出比原始数据更高的估测精度;3)在使用RFR方法的情况下,基于贡献度前10的特征因子和累积贡献度达到70%的特征因子建模时,经过ATC算法滤波后的时间序列数据均表现出了最优的估测精度;4)基于ATC算法和贡献度前10的特征因子对高山松碳储量的建模效果最优,其决定系数R2为0.867、均方根误差RMSE为15.527 t/ha、预测精度P为73.54%、相对均方根误差rRMSE为41.14%;4)基于最优估测模型的高山松碳储量反演结果分别为:677万吨(1987年)、716万吨(1992年)、722万吨(1997年)、436万吨(2002年)、720万吨(2007年)、711万吨(2012年)、753万吨(2017年)。使用ATC滤波算法能够有效去除高海拔地区时间序列影像中的噪声,从而降低时间序列影像的不确定性,提高了高山松碳储量遥感估测精度。
关键词:
Landsat时间序列 滤波 高山松 碳储量 ATCTime series remote sensing data has good applications in accurately estimating forest carbon storage, providing data support for a deeper understanding of the carbon cycle process of forest ecosystems, scientific management, and protection of forest resources, but there is a lot of noise in remote sensing time-series data, in order to enhance the accuracy of estimating carbon storage, a filtering algorithm was developed to reduce the interference of noise in Landsat time-series data from high-altitude areas. Based on the continuous inventory fixed plots data of National Forest Inventory in Shangri-La area for the years 1987, 1992, 1997, 2002, 2007, 2012, and 2017, as well as Landsat time series images from 1987 to 2017. In this study, the Adaptive Topography Convolution(ATC)algorithm was developed using Python, which takes into account the impact of terrain factors on image quality, removes the noise from the image while retaining as much detail as possible, and uses Savitzky Golay filtering and median filtering to filter Landsat time series data, using the Random Forest Regression (RFR) algorithm, a carbon storage estimation model for Pinus Densata in Shangri La City was constructed. The optimal estimation model was selected to invert and map the carbon storage of Pinus Densata in 1987, 1992, 1997, 2002, 2007, 2012, and 2017. The results showed that 1) According to the average absolute error (MAE) of the image quality evaluation index, the image quality is the best after filtering with the ATC algorithm. In addition, the PSNR value of the time series data filtered by the ATC algorithm is relatively high, indicating an improvement in data quality; 2) When using the random forest regression algorithm, the filtered data showed higher fitting and prediction accuracy than the original data; 3) When using the random forest regression algorithm, the time series data filtered based on the ATC algorithm has the best estimation accuracy when selecting the top 10 feature factors with contribution and the feature factors with cumulative contribution reaching 70% for modeling; 4) The estimation model constructed based on ATC filtered time series and remote sensing features (Number of features 10) and random forest algorithm has the best performance in research, with a determination coefficient R2 of 0.867, root mean square error RMSE of 15.527 t/ha, prediction accuracy P of 73.54%, and relative root mean square error rRMSE of 41.14%; 5) The carbon storage inversion results of Shangri La Pinus Densata based on the optimal estimation model are as follows: 6.77 million tons (1987), 7.16 million tons (1992), 7.22 million tons (1997), 4.36 million tons (2002), 7.20 million tons (2007), 7.11 million tons (2012), 7.53 million tons (2017). From the inversion results, it can be observed that during the period from 1987 to 1997, the carbon storage of Shangri-La Pinus Densata showed a gradually increasing trend. However, from 2002 to 2017, the carbon storage exhibited a significant fluctuating trend. The use of ATC filtering method can effectively remove noise in time series images of high-altitude areas, thereby reducing the uncertainty of time series images and improving the accuracy of remote sensing estimation of Pinus Densata carbon storage.