下载中心
优秀审稿专家
优秀论文
相关链接
首页 > , Vol. , Issue () : -
摘要
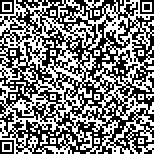
降低样本的依赖度,实现大区域、复杂作物类型的遥感监测识别是农业遥感的重要研究内容。本研究以黑龙江省产粮大市绥化为例,深入探索了样本数量对作物分类效果的影响,以及小区域尺度训练的监督分类模型空间外推至大区域尺度的可行性。研究发现,采用玉米播种至抽穗期中期的Sentinel-2时序遥感影像,在优化随机森林模型参数的基础上,当训练的样本量从10%逐步递增至50%时,即玉米、水稻和大豆的训练样本各为130个左右,就能提取北林区作物的空间分布,整体分类精度为94.6%,当样本量进一步增加时,模型的整体分类精度保持平稳,并不会进一步增长。因水稻在育秧期-淹水期的陆表水体等光谱指数与玉米、大豆的显著差异,采用玉米播种至拔节期前期的 Sentinel-2遥感影像,即可实现北林区高精度的作物遥感识别。当时间从玉米拔节期前期延长至抽穗中期,作物的总体分类精度仅有微小的提升。作物空间分布和概率分布图表明,将北林区训练的最优模型外推至整个绥化市时,能取得与用绥化市采集的样本直接训练的模型得到相似的分类效果,整体分类精度为93.7%,仅比后者低1.3个百分点。距离、样本的空间代表性和数量、小区域和目标拓展区的作物种植结构的相似性是影响模型空间外推效果的关键因子。不同的作物对距离的敏感程度不一,由于水稻的水体指数、短波红外1等波段与其他作物的显著差异,水稻的分类效果对距离的变化并不敏感,而玉米和大豆的分类效果则随着外推距离的增长,总体上呈现下降的变化趋势。在源区域和目标拓展区作物种植结构相似的前提下,小区域的作物分类模型构建,需要同步兼顾样本的空间代表性和数量,才能取得较好的模型空间外推效果。本研究的结果为大尺度区域作物的遥感精准分类提供了高效与经济的方法,为作物分类样本的采集与抽样策略的制定、分类时相、敏感波段的选择提供了科学依据,为鲁棒性和泛化能力更强的模型外推方法的设计提供了有意参考。
Reducing the reliance on in-situ crop type samples is critical for remotely sensed crop type classification over large areas. This study used Suihua, a major grain-producing city in Heilongjiang Province, as an example to investigate the effect of sample size on crop type classification and to test the possibility of extrapolating supervised classification models trained on a small region to a larger area. Specifically, this study trained the crop type classification model in Beijing District and then extrapolated it to the whole of Suihua. First, a parameter-optimized random forest model was trained and used to identify the spatial distribution of crops in Beilin District in 2022 using Sentinel-2 remote sensing imagery from sowing to mid-tasseling of maize. We found that the overall accuracy (OA) gradually increased as the proportion of samples participating in the random forest training increased from 10% to 50% of the GVG samples in Beilin District. The model achieved the best performance with a maximum OA of 94.6% when 50% of the GVG samples in Beilin District were used for crop classification, where maize, rice and soybean had approximately 130 training samples. Thereafter, the performance of the model remained stable even as the number of in-situ crop samples increased. We also found that the most important features in the classification of maize, soybean and rice were REP at the tassel stage of maize, SWIR1 at the pod stage of soybean and LSWI at the transplanting stage of rice. Secondly, we extrapolated the best trained model in Beilin District to classify crop types in the whole of Suihua. We found that the model extrapolation achieved an OA of 93.7% for crop type classification in Suihua, which was only 1.3% lower than the model trained directly in Suihua. The similarity of the spatial and probability distribution maps of the crops between the Beilin model and the Suihua model indicated that the extrapolation of the crop classification model in a small area can achieve a comparable classification result to the crop classification model trained directly in a large area. Finally, we carefully examined the effects of distance, spatial representativeness and number of samples, and similarity of crop structure between small area and target expansion area on model extrapolation. We found that different crops have different sensitivities to distance, and the classification effect of rice was insensitive to changes in distance due to significant differences between the LSWI and SWIR1 of rice and other crops, while the classification effects of maize and soybean show an overall decreasing trend of change with increasing extrapolation distance. In short, when building crop classification models in small regions with similar crop structures in both source and target areas, not only the number of samples should be considered, but also the representativeness of their spatial distribution. This would ensure that the model is adequately trained and can achieve a better spatial extrapolation effect. The results of the study provide a cost-effective and efficient method to accurately classify crops over large areas using remote sensing. In addition, the study provides a scientific basis for developing crop sampling strategies, selecting sensitive bands and determining the classification time window. It also provides a valuable reference for the development of model extrapolation methods with greater robustness and generalizability.