下载中心
优秀审稿专家
优秀论文
相关链接
首页 > , Vol. , Issue () : -
摘要
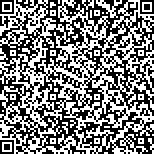
针对形状尺度多变和边界提取不够准确两个建筑物提取难题,提出一种集成主体边缘分离和多尺度信息提取的双分支建筑物提取网络。首先,利用解耦思想和光流技术,设计一种主体边缘分离分支,从而获取建筑物的主体和边缘特征,增强对建筑物边界的表征能力。然后,基于空洞卷积、深度可分离卷积和注意力机制,构建一种轻量级多尺度信息提取分支,来充分提取不同尺度的建筑物特征。最后,借助所获取的主体和边缘特征,给出一种主体和边缘特征辅助增强的损失函数,优化网络训练过程。两组常用公开建筑物提取数据集上的实验结果表明,所提出建筑物提取网络可行有效。
Objective Remote sensing(RS) image building extraction is one of the research hotspots in the field of RS, which is of great significance for urban planning, illegal building detection, natural disaster assessment and so on. With the rapid development of deep learning technology, it has been introduced into remote sensing image building extraction and achieved significant extraction effect. There are two main challenges for building extraction: 1) The buildings in RS images have different scales and shapes, and 2) it’s difficult to accurately extract building boundaries. Method To address the above two challenges, this paper proposes a two-branch building extraction network integrating main body and edge separation and multi-scale information extraction. First, a main body and edge separation branch (MBESB) is designed for feature decomposition based on the decoupling idea and optical flow estimation technique. MBESB generates the main body and edge features of buildings respectively, thereby enhancing the ability of representing the building boundaries. Then, to fully extract the different-scale features of buildings, a lightweight multi-scale information extraction branch is constructed based on dilated convolution, depth-separable convolution and attention mechanism. Finally, in order to improve the training process of building extraction network, a body-edge-feature-enhanced loss function is presented with the help of the generated main body and edge features. Result Experiments were carried out with two public building extraction datasets, namely the Inria and WHU datasets, in order to evaluate the performance of the proposed MMT-Net method. Five deep learning methods were used as the comparative methods. Quantitative analysis of building extraction results was done on four evaluation metrics, namely precision, recall, F1, and IoU. For the Inria and WHU datasets, the F1/IoU values of the proposed MMT-Net are 0.8894/0.8008 and 0.9567/0.9170, respectively, which are superior to the five comparative methods. Conclusion Experimental results on two commonly used public building extraction datasets show that the proposed building extraction network is feasible and effective. In addition, the ablation experiments’ results indicate that all of the MBESB, the LMIEB and the loss function with auxiliary enhancement of the main body and edge features proposed in this work can enhance the building extraction performance effectively.