下载中心
优秀审稿专家
优秀论文
相关链接
首页 > , Vol. , Issue () : -
摘要
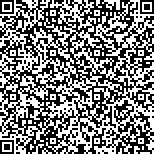
明确山区季节性积雪的时空变化对山区水资源管理、水文过程和生态保护至关重要,高时空分辨率的积雪面积数据是监测山区积雪变化的重要手段。然而现有的积雪面积遥感产品,受传感器性能限制,高时间和高空间分辨率无法兼得,难以准确捕捉高度异质的山区斑状积雪的细微变化。本研究选取祁连山北麓山区,利用MODIS和Landsat-8两种卫星数据,发展了基于U-Net++网络多源数据融合获取高时空分辨积雪面积的方法,使用高分辨率的Landsat数据和Sentinel数据对新发展的方法进行验证,并与融合高时空反射率数据间接重建积雪面积的STARFM和DMNet时空融合算法从不同角度进行对比验证。验证结果表明:(1)基于U-Net++网络的30 m空间分辨率积雪面积重建算法有效恢复了山区积雪的细节特征。算法精度较高,总体精度为90.4%,制图精度为89.9%,用户精度为88.4%,Kappa系数为0.80。重建结果鲁棒性较好,在不同积雪覆盖度、不同地表下垫面和不同云量的条件下,总体精度相差小于3%,且均高于88%。(2)相较于STARFM和DMNet时空融合间接重建积雪面积的方法,本算法总体精度提高了8.7%和5.2%,制图精度提高了24.1%和18.4%,Kappa系数提高了0.20和0.12。总体而言,本研究开发的基于U-Net++网络的30m空间分辨率逐日积雪面积重建算法,可以有效重建高精度高时空分辨率积雪面积数据,对山区径流模拟、积雪水储量估算和灾害防治等具有重要意义。
Abstract: The abstract of this study contains four sections objective, method, result and conclusion: Objective: High spatial and temporal resolution snow cover remote sensing range data is the key driving data for hydrological simulation. The accuracy of snow cover range directly determines the reliability of simulation results. Existing snow range remote sensing products, limited by sensor performance, often cannot achieve both high temporal and spatial resolution, and are stretched when facing highly spatially heterogeneous patchy snow distribution. As an important driving data for hydrological simulation, daily snow cover extent data with a spatial resolution of 30 m is crucial to improving the accuracy of basin-scale hydrological simulation. The purpose of this study is to prepare 30m daily high-precision snow coverage data in the Qilian Mountains. Method: This study used the U-Net++ deep learning network to predict the snow cover range with high spatiotemporal resolution. First, the Landsat-8 SR reflectance data at the previous time t1 and the MOD09GA reflectance at t1 and t2 were resampled and the pixels were matched. L1’, M1’ and M2’ were obtained by calculating NDSI, NDVI and NDFSI index data for the three images. M1’ - M2’ and L1’ were used as feature inputs to the U-Net++ network. The snow range obtained from the Landsat-8 SR reflectance at time t2 by the snow accumulation recognition algorithm was used as the network training truth value. Finally, any two moments corresponding to the features of the network were input, and the network reconstructed the high-resolution snow extent data corresponding to the latter moment. Result: The reconstruction result based on the U-Net++ algorithm effectively restored the snow range information under the complex underlying surface. The overall accuracy verified in the northern foothills of the Qilian Mountains was 90.490.9%, the producer accuracy was 89.990.2%, and the user accuracy was 88.488.6 %, the Kappa coefficient was 0.8040.814, which is almost completely consistent with the real snow range. Among different snow coverages, the overall accuracies of low, medium and high coverage are 89.2689.95%, 92.9192.64% and 90.5589.26%. Among different land surface covers, the overall accuracy of the bare soil area was 91.2091.69% and the overall accuracy of the vegetation area was 89.0589.91%. It was compared with spatio-temporal fusion algorithms that indirectly reconstruct snow cover information based on satellite data fusion reflectivity such as STARFM and DMNet networks. The overall accuracy increased by 8.7% and 5.2% respectively. Conclusion: The snow coverage reconstruction method based on U-Net++ network is suitable for the reconstruction of snow coverage data with high spatial and temporal resolution. The snow coverage range data reconstructed based on this method in the northern foothills of the Qilian Mountains has high accuracy, and has good reconstruction results at different snow coverage levels and different surface types. It has good reconstruction results for large areas of snow in high coverage areas and patchy snow in medium and low coverage areas. It has good reconstruction results for bare soil areas and vegetation areas and has strong stability.