下载中心
优秀审稿专家
优秀论文
相关链接
首页 > , Vol. , Issue () : -
摘要
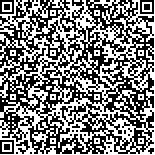
高时空分辨率雪深数据对水文建模和灾害预报至关重要。目前,高时间分辨率雪深通常源于被动微波数据,而其较粗的空间分辨率还无法满足区域水文和灾害研究的需求。本研究基于被动微波和光学等多源遥感数据,提出一种耦合深度学习模型(FT-Transformer)与积雪微波辐射传输模型(Snow Microwave Radiative Transfer, SMRT)的降尺度雪深反演算法。使用深度学习映射AMSR 2亮温差(Brightness Temperature Deviation, TBD)、积雪覆盖日数)Snow Cover Days, SCD)和积雪面积比例(Snow Cover Fraction, SCF)等特征与雪深的复杂非线性关系,耦合SMRT拟合等效雪粒径(Effective Snow Grain size, ESG)实现降尺度反演雪深,使用北疆39个站点数据进行模型训练和验证,获取了北疆500 m降尺度雪深。验证结果表明:引入SCD独立验证RMSE优化了18%,有助于提高模型的空间泛化能力;ESG显著优化了深度学习反演雪深的精度,RMSE为6.82 cm,较未添加ESG的模型提升了15%,同时大幅度改进了深雪低估。进行时间序列分析,发现ESG符合实测雪深的变化趋势,能够约束优化模型反演雪深的稳定性。最后对比已有的AMSR 2、ERA5-Land和SDDsd雪深产品,本研究降尺度雪深精度最优,RMSE为6.51 cm,雪深空间分布也更为精细,体现了山区复杂的雪深异质性。本研究探索了积雪微波辐射传输模型结合深度学习在降尺度雪深反演的可行性,为获取区域降尺度雪深产品提供了保障。
High spatiotemporal resolution snow depth data is crucial for hydrological modeling and disaster forecasting. Currently, high temporal resolution snow depth data is typically derived from passive microwave measurements, but its coarser spatial resolution cannot meet the needs of regional hydrology and disaster research. Based on passive microwave brightness temperature data and combined with high-resolution optical remote sensing data, this paper aims to develop a high-precision downscale snow depth inversion algorithm to provide high spatial and temporal resolution snow depth data for regional-scale hydrology and climate research. This study proposes a downscaling snow depth retrieval algorithm based on multi-source remote sensing data such as passive microwave and optical, coupled with a deep learning model (FT Transformer) and a snow microwave radiative transfer (SMRT) model. Deep learning is used to map the complex nonlinear relationship between features such as AMSR 2 Brightness Temperature Deviation (TBD), Snow Cover Days (SCD) and Snow Cover Fraction (SCF) and snow depth, At the same time, considering the influence of the physical properties of snow, the coupled SMRT is fitted with the effective snow grain size (ESG) to characterize the spatiotemporal dynamic snow properties, and is input into the deep learning model to achieve downscale inversion of snow depth. This algorithm was used to obtain downscaled snow depth data at 500 m spatial resolution in northern Xinjiang.Model training and validation were conducted using observed data from 39 stations in northern Xinjiang. The validation results revealed that Snow Cover Days (SCD) can effectively represent the snow accumulation process. Independent validation showed an 18% improvement in RMSE, indicating enhanced spatial generalization capability of the model. The inclusion of the Effective Snow Grain (ESG) feature significantly improved the overall accuracy of the deep learning-based downscaled snow depth retrieval, resulting in an RMSE of 6.82 cm. This represents a 15% improvement compared to the model without the ESG feature. Additionally, the inclusion of the ESG feature greatly mitigated the underestimation of deep snow (>40 cm), leading to a 35% improvement in RMSE for such conditions. Furthermore, a time series analysis of the snow depth retrieval using the ESG feature demonstrated that it aligns with the observed snow depth variations, thereby constraining and stabilizing the output of the FT-Transformer model. Finally, when compared to existing snow depth products such as AMSR2, ERA5-Land, and SDDsd, the downscaled snow depth data from this study exhibited superior validation accuracy, with an RMSE of 6.51 cm. The spatial distribution of snow depth was also more refined, particularly capturing the complex snow depth heterogeneity in the mountainous regions of northern Xinjiang. This study explored the feasibility of combining the Snow Microwave Radiative Transfer (SMRT) model with deep learning for downscaled snow depth retrieval, It has obtained a downscaled snow depth product with high accuracy performance in northern Xinjiang, providing assurance for the demand of high spatiotemporal resolution snow depth data at the regional scale.