下载中心
优秀审稿专家
优秀论文
相关链接
首页 > , Vol. , Issue () : -
摘要
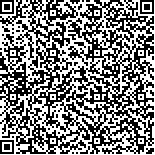
高效准确地获取高空间分辨率的浅海水深能够为海上航运、海洋资源普查与保护等提供数据支持。本文提出了一种引入地理位置特征作为建模要素的水深反演方法,构建了基于BP神经网络的水深反演模型。分别利用Sentinel-2、Landsat-9等不同的遥感影像,在中国涠洲岛海域和美国莫洛凯岛海域对本文提出的方法进行精度和适用性检验。结果表明:在模型筛选过程中发现,机器学习模型建模精度高于所有经验模型,其中BP神经网络模型建模精度最高。引入地理位置特征后可以很好地提高水深反演精度。经过实验验证,涠洲岛地区反演精度R2从0.7666提升到0.9952,RMSE从2.5016m减小到0.3578m。作为验证实验的莫洛凯岛地区R2也达到了0.9939,RMSE从3.0165m减小到1.0189m。表明本文构建的水深反演模型精度高、可靠性强、可移植性好,可以有效地用于浅海测深。在加入地理位置特征后,同时加入植被指数特征并没有取得更好的结果,反而使模型的建模精度略有降低,说明盲目地增加建模要素并不能提高模型精度,应分析各要素之间的自相关性,进行综合分析取舍建模因子。
Bathymetric maps with high spatial resolution can display topographic details and provide data support for maritime navigation, coastline management, and marine resource utilization and development. This study conducted experiments Weizhou Island, China, and Molokai Island, USA sea areas. With the support of Sentinel-2and Landsat-9 images, a water depth inversion method was proposed, which incorporates geographic location features as modeling elements, and an optimal water depth inversion model based on a BP neural network was constructed. Finally, different remote sensing data were used to conduct accuracy tests of the inversion method proposed in this paper in various sea areas. The results indicate that: During the model selection process, it was found that machine learning models demonstrated higher modeling accuracy than all empirical models.The BP neural network model exhibits the highest modeling accuracy in machine learning models. In addition, the machine learning model is more stable, the inversion of the water depth map can better invert the actual water depth change in the experimental area, and the inversion image is smoother. The introduction of geographic location features can significantly improve the accuracy of water depth inversion. Experimental results have shown that the inversion accuracy in the Weizhou Island was improved from an R2 value of 0.7666 to 0.9952 and the RMSE was reduced from 2.5016m to 0.3578m. As a validation experiment, the R2 value in the Molokai Island area was 0.9939, and the RMSE decreased from 3.0165m to 1.0189m. At the same time, the introduction of geographic location features can also eliminate the influence of some clouds and fog on remote sensing images, and obtain more accurate water depth inversion results. The conclusion demonstrated that using all bands of the image for modeling, the inversion of the water depth map is smoother, and better able to invert regional bathymetry trends, with fewer outliers and more accurate inversion results. After incorporating geographic location features, the addition of vegetation index features did not yield better results. Instead, it slightly decreased the modeling accuracy of the model. This indicates that blindly adding modeling elements does not necessarily improve modeling accuracy. Analyzing the autocorrelation between each element and making comprehensive decisions on modeling factors is important. In summary, the water depth inversion model constructed in this paper has high accuracy, strong reliability, and good portability, and can be effectively used for shallow sea depth measurement.