下载中心
优秀审稿专家
优秀论文
相关链接
首页 > , Vol. , Issue () : -
摘要
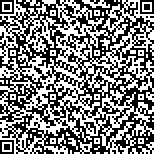
地面沉降预测对于城市地面沉降模式的深入分析和早期预警具有重要的指导意义。传统的数值预测模型在捕捉地面沉降数据复杂特征方面存在一定困难,导致预测结果的准确性不高。为了解决这一问题,本研究基于SBAS-InSAR方法获取的新密市地面沉降信息,构建了结合趋势和季节特征长短时记忆网络的地面沉降预测模型(TSC-LSTM,Trend Seasonal Characteristics-LSTM),该模型联合基于加权回归季节趋势分解(STL)在沉降数据时序特征提取方面的优越性和长短期记忆模型(LSTM)在时序预测方面对梯度消失问题的处理优势,实现对地面沉降数据更准确的预测。结果表明:(1)新密市2018年到2022年的地面沉降速率为-60.3 ~ 51.96 mm/a,共形成五个地面沉降中心区域。其中,最大累计沉降和最大累积隆起分别为304.9 mm和197.68 mm。(2)TSC-LSTM模型在五个沉降中心区域的预测中表现出色,TSC-LSTM模型的R2值范围为0.9985 ~ 0.9992,明显高于次优模型LSTM的0.9662 ~ 0.9872。TSC-LSTM模型预测精度的RMSE值小于2 mm,达到了1.2426 ~ 1.7403 mm。(3)单点预测结果表明,TSC-LSTM模型能够更精确的把握累积沉降数据中的局部变化趋势。本研究提出的方法能为城市地面沉降的深入研究提供有力支持。
The prediction of ground subsidence is of great significance for the in-depth analysis and early warning of urban ground subsidence patterns. Traditional numerical prediction models face difficulties in capturing the complex features of ground subsidence data, leading to low accuracy in prediction results. To address this issue, this study, based on the SBAS-InSAR method, obtained ground subsidence information in Xinmi City and constructed a ground subsidence prediction model (TSC-LSTM, Trend Seasonal Characteristics-LSTM) that combines trend and seasonal features with long short-term memory networks. The model leverages the advantages of weighted regression seasonal trend decomposition (STL) in extracting temporal features of subsidence data and the handling of the vanishing gradient problem by the long short-term memory model (LSTM) in temporal prediction. The results show that (1) The ground subsidence rate in Xinmi City from 2018 to 2022 ranges from -60.3 to 51.96 mm/year, forming five ground subsidence centers. The maximum cumulative subsidence and maximum cumulative uplift are 304.9 mm and 197.68 mm, respectively.(2) The TSC-LSTM model performs well in the prediction of subsidence in the five centers, with R2 values ranging from 0.9985 to 0.9992, significantly higher than the suboptimal LSTM model with a range of 0.9662 to 0.9872. The RMSE values for the TSC-LSTM model"s prediction accuracy are less than 2 mm, ranging from 1.2426 to 1.7403 mm.(3) Single-point prediction results indicate that the TSC-LSTM model can more accurately capture local variations in the cumulative subsidence data trends. The proposed method in this study provides strong support for the in-depth research of urban ground subsidence.