下载中心
优秀审稿专家
优秀论文
相关链接
首页 > , Vol. , Issue () : -
摘要
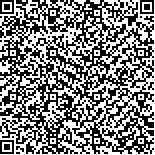
红树林是最富有生物多样性、生产力最高的海洋生态系统之一,整合高分辨率遥感影像和深度学习的红树林群落精细分类已成为当前研究的热点和难点。本文提出一种新颖的深度学习分类网络模型SSAFormer(Swin-Segmentation-Atrous-Transformer)进行红树林群落精细分类。该模型以视觉变压器的变体Swin Transformer为主干网络,在主干网络加入了CNN(Convolutional Neural Network)架构中的空洞空间卷积池化金字塔ASPP(Atrous Spatial Pyramid Pooling)提取更多尺度特征信息,在轻量级解码器中嵌入了特征金字塔FPN(Feature Pyramid Network)结构来融合低层和高层丰富的语义特征信息。本文利用GF-7(Gaofen-7)多光谱影像和UAV-LiDAR点云构建了三种主被动特征数据集,并对比分析SegFormer和本文改进的Swin Transformer算法的分类结果,进一步论证提出的SSAFormer算法对红树林群落的分类性能。研究结果表明:(1)相比于SegFormer和改进的Swin Transformer算法,SSAFormer实现了红树林的精细分类,总体精度OA(Overall Accuracy)提高了1.77%~5.3%,Kappa系数最高为0.8952,平均用户交并比MIou(Mean Intersection over Union)最大提升了7.68%;(2)在GF-7多光谱数据集上,SSAFormer算法实现了91%最高总体精度(OA),在UAV-LiDAR数据集上SSAFormer算法平均用户交并比(MIou)提升至57.68%,在加入光谱特征的UAV-LiDAR数据集上,SSAFormer算法平均用户交并比(MIou)的均值提高了1.48%;(3)UAV-LiDAR数据相比于GF-7多光谱数据平均用户交并比(MIou)最大提高了5.35%,总体精度(OA)的均值提升了1.81%,加入光谱特征的UAV-LiDAR数据分类精度(F1-score)提高了2.6%;(4)提出的SSAFormer算法实现了海榄雌分类精度(F1-score)97.07%最高,桐花树分类精度(F1-score)达到91.99%,互花米草分类精度(F1-score)达到93.64%,桐花树的分类精度(F1-score)的平均值在SSAFormer模型上达到了86.91%最高。上述结论证明了本文所提出的模型能够有效提高红树林群落分类精度。
Abstract: (Objective) Mangroves are one of the most biodiverse and productive marine ecosystems, and the fine classification of mangrove communities by combining high-resolution remote sensing images and deep learning has become a hot and difficult topic in current research. (Methods) In this paper, we proposed a novel deep learning classification network model SSAFormer (Swin-Segmentation-Atrous-Transformer) for fine classification of mangrove communities. The SSAFormer used Swin Transformer, a variant of Visual Transformer, as the backbone network. The Atrous Spatial Pyramid Pooling (ASPP) in the Convolutional Neural Network (CNN) architecture was added to the backbone network to extract more scale feature information. The Feature Pyramid Network (FPN) structure was embedded in the lightweight decoder to fuse the rich semantic feature information of the low and high layers. In this paper, three active and passive feature datasets were constructed based on GF-7 multispectral imagery and UAV-LiDAR point clouds, and the classification results of the improved Swin Transformer and SegFormer algorithms were compared and analyzed to further demonstrate the classification performance of the SSAFormer algorithm for mangrove communities. (Result) The results of the study revealed that:(1) Compared with the improved Swin Transformer and SegFormer algorithms, SSAFormer achieved a fine classification of mangroves, with an overall accuracy (OA) increase of 1.77%~ 5.3%, Kappa up to 0.8952, and a mean intersection over union (MIou) was improved by 7.68%;(2) On the GF-7 multispectral dataset, the SSAFormer algorithm achieved the highest overall accuracy (OA) of 91%, and the mean intersection over union (MIou) of the SSAFormer algorithm on the UAV-LiDAR dataset improved to 57.68% on the UAV-LiDAR dataset with the inclusion of spectral features. The mean value of the SSAFormer algorithm mean intersection over union (MIou) improved by 1.48%;(3) The UAV-LiDAR data showed a maximum improvement of 5.35% in the mean intersection over union (MIou) compared to the GF-7 multispectral data, a mean improvement of 1.81% in the overall accuracy(OA), and an improvement of 2.6% in the classification accuracy (F1-score) of the UAV-LiDAR data with the inclusion of spectral features;(4) Based on the SSAFormer algorithm, the highest classification accuracy (F1-score) of 97.07% was achieved for Avicennia marina, the classification accuracy (F1-score) of Aegiceras corniculatum achieved 91.99%, the classification accuracy (F1-score) of Sporobolus alterniflorus reached 93.64%, and the average value of classification accuracy (F1-score) of Aegiceras corniculatum reached the highest 86.91% on SSAFormer model. (Conclusion) The above conclusions proved that the proposed model can effectively improve the classification accuracy of mangrove communities.