下载中心
优秀审稿专家
优秀论文
相关链接
首页 > , Vol. , Issue () : -
摘要
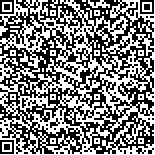
高光谱遥感在水质监测方面具有巨大潜力,目前多集中在光学特性水质参数定量反演研究,而对同样能够反映人类活动对水体影响的非光学水质参数的研究相对较少。基于此,本文选择广东省某城中村河流作为研究区,开展了无人机高光谱遥感同步水体样本数据采集实验,提出了一种基于LBFGS加速多层感知网络模型(LBFGS-MLP)对城市河流的非光学特性水质参数进行反演研究。首先,通过Pearson相关分析筛选出总磷、总氮、氨氮三种非光学特性水质参数的相关波段作为模型输入;然后,在探究不同网络层数和优化算法对模型性能影响的基础上,基于LBFGS优化算法对多层感知网络进行加速;最后,利用构建的LBFGS-MLP模型应用于研究区水体的非光学特性水质参数浓度空间分析。总体而言,在总磷、总氮和氨氮三种非光学特性水质参数浓度的训练数据集和测试数据集上,提出的LBFGS-MLP模型反演精度明显优于随机森林、CatBoost和XGBoost模型,尤其在总氮和氨氮浓度的反演上;模型的决定系数(R2)分别为0.71、0.82和0.72,平均绝对误差(MAE)分别为0.0118、0.0394和0.0601mg/L;研究区内总磷浓度主要分布在0.1~0.3mg/L之间,总氮浓度在2~5mg/L之间,氨氮浓度在0.1~0.4mg/L之间,与调查结果一致。通过本文的研究,验证了多层感知网络在非光学特性水质参数反演的有效性和可行性,可为更全面地评估城市河流水体状况提供理论依据和参考。
Water is the source of life, the foundation of survival, the necessity of production, and the basis of ecology. However, under the dual pressures of human activities and climate change, aquatic ecosystems are facing increasingly severe challenges, especially the serious problem of water pollution, which directly threatens the physical and mental health of residents. Water quality monitoring plays a crucial role in water pollution control, which precisely evaluates the health of water bodies and promptly adjusts control strategies, ensuring the stability and health of water environmental quality. Hyperspectral remote sensing holds significant potential in water quality monitoring, and with the rapid development of unmanned aerial vehicles (UAV) and hyperspectral technology, UAVs equipped with hyperspectral sensors have greatly improved in spectral resolution and spatial resolution. This makes water quality parameter inversion using hyperspectral remote sensing gradually become a research hotspot. However, current research predominantly focuses on optical water quality parameters, with relatively less emphasis on non-optical parameters that also reflect human activities" impact on water bodies. In this study, an urban river in a certain village in Guangdong Province is chosen as the study area, and an experiment is conducted involving UAV for hyperspectral remote sensing image acquisition and simultaneous water sample collection. Then, we propose a multilayer perceptron (MLP) network model accelerated by limited-memory Broyden-Fletcher-Goldfarb-Shanno (LBFGS), named LBFGS-MLP for the inversion of non-optical water quality parameters. The parameters include total phosphorus (TP), total nitrogen (TN), and ammonia nitrogen (NH3-N), which are important indicators for measuring the nutritional status of water bodies. Specifically, through Pearson correlation analysis, spectral bands related to the three non-optical water quality parameters of TP, TN, and NH3-N, are selected as model inputs. Subsequently, based on exploring the impact of different network depths and optimization algorithms on model performance, the LBFGS optimization algorithm is adopted to accelerate the multi-layer perceptron network, and the loss function is mean squared error (MSE). Finally, the LBFGS-MLP model is applied to spatially analyze the concentrations of TP, TN, and NH3-N in the study area. Overall, the LBFGS-MLP model demonstrates significantly better accuracy on both training and testing datasets for the concentrations of TP, TN, and NH3-N compared to the Random Forest, CatBoost, and XGBoost models, particularly in the inversion of TN and NH3-N concentrations. The model"s coefficients of determination (R2) are 0.71, 0.82, and 0.72, and the mean absolute errors (MAE) are 0.0118, 0.0394, and 0.0601 mg/L, respectively. The concentrations of TP, TN, and NH3-N in the study area are mainly distributed between 0.1~0.3 mg/L, 2~5 mg/L, and 0.1~0.4 mg/L, respectively, consistent with the survey results. Through this study, the effectiveness of the MLP algorithm in the inversion of non-optical water quality parameters is verified, providing a theoretical basis and reference for a more comprehensive assessment of the urban river water body condition.