下载中心
优秀审稿专家
优秀论文
相关链接
首页 > , Vol. , Issue () : -
摘要
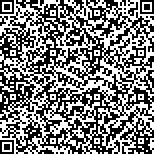
高标准农田建设是提高我国粮食生产能力和保障粮食安全的关键举措。其中,农村(高标准农田)道路,例如田间道和生产路,是高标准农田基础设施建设的核心内容之一。如何利用高分辨率遥感卫星影像快速准确地提取农村道路,对于高标准农田建设的监测与监管具有重要意义。近年来,针对城市道路提取的深度学习方法与基准数据集发展迅猛,但受限于城市道路与农村道路的巨大分布差异,面向城市道路提取的训练模型难以直接应用于农村道路提取任务。本文从基准数据集出发,系统构建了首套高分遥感影像农村(高标准农田)道路提取基准数据集(WHU-RuR),覆盖我国华北、华中、华东、西北和西南的七省的典型农村区域(湖北省、湖南省、陕西省、四川省、安徽省、河南省和河北省),包含训练集与测试集共25922对高分遥感影像与道路样本(空间分辨率0.3m,空间尺寸1024×1024)。本数据集为目前农田覆盖率最高、农村道路类别最多、农村背景最复杂以及数据量最大的开源数据集。同时,为了验证WHU-RuR数据集的可用性,本文测试并全面分析了前沿深度学习道路提取方法在农村道路提取任务上的效果。实验表明,WHU-RuR数据集可基本满足农村道路提取需求,在高标准农田监测与监管领域具有重大应用潜力。数据集链接:https://doi.org/10.57760/sciencedb.09181。
High-standard farmland construction is the crucial initiative in enhancing grain production capacity and ensuring food security in China. Rural roads, including field paths and production routes, are the core components of the infrastructure on high-standard farmland construction. The rapid and accurate extraction of rural roads from high-resolution satellite imagery is of significant importance for monitoring and regulating high-standard farmland construction. Although deep learning methods and benchmark datasets for urban road extraction had seen rapid development in recent years, trained models faced urban road extraction are difficult to apply directly to rural road extraction tasks, due to the substantial distribution differences between urban and rural roads. Starting from a benchmark dataset, we systematically constructed the first benchmark dataset for rural (high-standard farmland) road extraction from high-resolution satellite imagery (WHU-RuR) in this paper. The WHU-RuR covers typical rural areas from seven provinces (Hubei, Hunan, Shaanxi, Sichuan, Anhui, Henan, and Hebei) of North China, Central China, East China, Northwest China, and Southwest China, and includes 25,922 pairs of high-resolution satellite images and road samples (spatial resolution of 0.3m, spatial size of 1024×1024) both in training and testing sets. Currently, the WHU-RuR is the most extensive open-source dataset with the highest farmland coverage, the greatest variety of rural road categories, the most complex rural backgrounds, and the largest data volume. Finally, in order to validate the utility of the WHU-RuR dataset, comprehensive analyses of state-of-the-art deep learning road extraction methods on rural road extraction tasks are conducted. The results indicated that WHU-RuR dataset satisfied the basic requirements for rural road extraction and has significant potential applications in monitoring and regulation field of high-standard farmland. The dataset can be accessed at: https://doi.org/10.57760/sciencedb.09181.