下载中心
优秀审稿专家
优秀论文
相关链接
首页 > , Vol. , Issue () : -
摘要
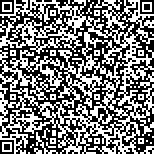
高光谱遥感时序精准监测湿地植被和水体状况已成为准确评估和全面监测岩溶湿地生态系统状况的重要基础。然而,现有星载高光谱影像的空间分辨率较低,难以捕捉湿地植被复杂的空间细节,已有超分辨率重建方法无法实现卫星和无人机跨平台影像重建,且难以实现对大范围湿地场景的时序监测。为了解决上述问题,本文提出一种跨平台的多尺度影像特征映射模块CMIFM(Cross-Sensor Multiscale Image Feature Mapping Module)。该模块统一了无人机高光谱影像AHSI(Aerial hyperspectral image)和星载高光谱影像SHSI(Spaceborne hyperspectral image)的空间尺度,根据携式地物光谱仪ASD(Analytical Spectral Devices)的实测数据将AHSI和SHSI映射在同一个光谱特证空间中,进而整合AHSI和SHSI的空谱特征信息,构建影像特征数据集。通过使用超分辨率网络(ESRGAN和SwinIR)实现SHSI的高质量影像重建。同时,本文采用最新的深度学习(DATFuse)和传统的融合方法(GS),对比在湿地场景下,Sentinel-2和OHS-02重建影像和融合影像中植被群落和水体的光谱和空间质量。研究结果表明:(1)基于CMIFM的超分辨率网络能够通过学习AHSI特征,实现跨平台提升SHSI中湿地植被和水体的空间纹理细节信息,在视觉感知和定量指标方面均优于GS影像融合方法,重建影像的平均PSNR和SSIM精度分别为11.06和0.3102;(2)重建影像中狗牙根、华克拉莎和芒草3种典型湿地植被群落以及水体的光谱特征和实测ASD数据相比具有高稳定性和保真性,且光谱波段的平均RMSE和R2精度值高于DATFuse和GS融合影像;(3)CMIFM+ESRGAN和CMIFM+SwinIR方法在空谱重建性能上具有较强的泛化能力,能够在AHSI未覆盖的区域中完成影像的重建,平均PSNR和R2分别为12.74和0.1897,接近AHSI覆盖区域的精度数值范围。(4)本文验证了基于CMIFM模块的超分辨率技术在复杂湿地高光谱影像重建中的可行性。
Time-series accurate monitoring of vegetation and water conditions by hyperspectral remote sensing is the key and foundation for accurate assessment and comprehensive monitoring of karst wetland ecosystem. However, the spatial resolution of the existing satellite hyperspectral images is low, which could hardly capture the complex spatial details of the wetland vegetations, while the super-high-resolution UAV images could hardly realize the time-sequence monitoring of the large-scale wetland scenes. The existing fusion methods could not well realize the non-destructive fusion of the spatial and spectral features of the hyperspectral images from the above two kinds of platforms. In order to solve the problems, this paper propose a cross-platform multi-scale image feature mapping module (Cross-Sensor Multiscale Image Feature Mapping Module, CMIFM). This module unifies the spatial scale of UAV hyperspectral image (Aerial hyperspectral image, AHSI) and satellite hyperspectral image (Spaceborne hyperspectral image, SHSI), maps AHSI and SHSI into the same spectral characteristic space according to the measured ASD (Analytical Spectral Devices) data, integrates the spatial- and spectral- feature fusion data of AHSI and SHSI to construct the image feature datasets. The high-quality image reconstruction of SHSI could be achieved by training feature datasets into super-resolution networks (ESRGAN and SwinIR). Meanwhile, this study used the latest deep-learning (DATFuse) and traditional (GS) fusion methods to compare the spatial- and spectral- quality of vegetations and water between the reconstructed and fused images in wetland scenes. This study highlights that: (1) CMIFM-based super-resolution network could realize cross-platform enhancement of spatial characteristics of detail information for wetland vegetation and water in SHSI by learning AHSI features, which could outperform the GS image fusion method in visual perception and quantitative indexes, and the average PSNR and SSIM accuracies of the reconstructed images are 11.06 and 0.3102, respectively. (2) the spectral features of three typical wetland vegetation communities (Cynodon-dactylon, Cladium chinense Nees and Miscanthus) and wetland water in the reconstructed images exhibit higher stability and fidelity based on the measured ASD data, and the average RMSE and R2 accuracies of the spectral bands are higher than the DATFuse and GS fusion images. (3) the CMIFM+ESRGAN and CMIFM+SwinIR methods provide strong generalization ability in terms of spatial- and spectral- reconstruction performance, and could be able to complete the reconstruction of the image in wetland scenes where AHSI is not covered, with the average PSNR and R2 of 12.74 and 0.1897, respectively, which are close to the range of accuracies’ values for the AHSI-covered area. (4) this paper verified the feasibility of CMIFM based super-resolution technology in hyperspectral reconstruction images of complex wetlands.