下载中心
优秀审稿专家
优秀论文
相关链接
首页 > , Vol. , Issue () : -
摘要
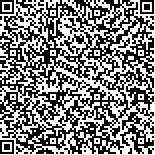
利用“深度学习+遥感”在高分遥感影像上快速识别滑坡,对于灾后应急救援工作的开展具有非常重要的现实意义。但目前为止,公开的深度学习滑坡识别数据集较少,难以满足相关研究人员利用深度学习方法开展滑坡识别研究的任务需求;基于此,本文使用谷歌地球影像和高分6号遥感影像制作并公开了一个样本数量达到10000+,空间分辨率为2米的深度学习滑坡数据集,用于相关学者开展研究。此外,为有效的识别滑坡,提取滑坡的边界及细节等信息,本文提出了一种改进的DeepLabV3+模型用于滑坡识别;即在DeepLabV3+模型的基础上,引入通道注意力机制特征融合模块和转置卷积来重构模型;实验结果表明,与主流算法(FCN, U-Net, SegNet, DeepLabV3+)相比,改进的DeepLabV3+模型在滑坡的边界及细节方面提取效果更好,所得结果更接近于真实标签。本文的相关代码和数据可在(https://github.com/ZhaoTong0203/landslides_identification_model_code.git)获得。
It is of great practical significance for post-earthquake emergency rescue to use the method of "Deep learning + Remote sensing" to quickly identify landslides from high-resolution remote sensing images after the earthquake. However, an excellent deep learning model can not be separated from a large-scale, high-quality data set as a support, the size of the data set and the quality of the target category data annotation information contained in it directly affect the performance and application effect of the deep learning model. So far, there are few public data sets of deep learning landslide identification, which is difficult to meet the task requirements of relevant researchers using deep learning methods to carry out landslide identification research. Based on this, this paper uses the visual interpretation method to annotate the pixel level of Gaofen-6 remote sensing image and Google Earth image taken after the earthquake, and uses the digital elevation model and optical image data to establish a three-dimensional model to assist, in order to ensure the accuracy of landslide annotation; Finally, a public deep learning landslide dataset with spatial resolution of 2m is established, which can be used to train deep learning semantic segmentation model and target detection model. The dataset contains 11581 groups of data, among which the training set contains 9265 groups of data, the validation set contains 1158 groups of data, and the test set contains 1158 groups of data, which far exceeds the data volume of the existing public landslide data set and basically meets the training data volume requirements of most deep learning landslide identification tasks. In addition, in order to recognize the boundary and detail information of landslides better and improve the accuracy of landslide recognition, this paper proposes an improved DeepLabV3+ landslide recognition model based on DeepLabV3+ network by introducing channel attention mechanism, feature map fusion module and transposed convolution; The feature map fusion module of the channel attention mechanism is used to adjust the weight between different feature map channels in the model training process, so as to effectively fuse the low-order features and high-order features output by the coding structure; and the transposed convolution uses a learnable convolution kernel to upsample the feature map, so as to better process complex image structure and semantic information. In the process of model training, this paper uses the transfer learning method to transfer the backbone network architecture and its parameters of the ResNet50 model trained on the ImageNet dataset to the encoder structure of the DeepLabV3+ network model to accelerate the training of the model. Experimental results show that, compared with the mainstream algorithms (FCN, U-Net, SegNet, DeepLabV3+), the improved DeepLabV3+ model has better extraction effect on the boundary and details of the landslide, and the results are closer to the real label; among them, MIOU is 87. 24%, Recall is 92. 47%, Precision is 90. 35, F1 score is 90. 87%, and Pixel Accuracy is 98. 91%. The code and data for this article are available at(https://github.com/ZhaoTong0203/landslides_identification_model_code.git). This research provides robust support for the advancement of deep learning in landslide identification and offers substantial practical assistance for post-earthquake emergency rescue efforts.