下载中心
优秀审稿专家
优秀论文
相关链接
首页 > , Vol. , Issue () : -
摘要
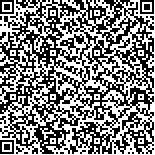
受成像环境、传感设备等因素的限制,高分辨率卫星遥感影像存在云干扰的现象,其严重影响了影像质量及后续的智能解译。深度学习因其强大的特征学习能力,近年来在在遥感影像去云中得到了广泛应用。针对现有遥感影像去云方法往往难以更好地保留图像边缘纹理细节的问题,本文提出一种基于注意力机制的生成对抗网络去云算法。首先设计傅里叶残差模块同时集成云层影像的低频和高频残差信息;然后利用注意力机制从通道和空间两个角度进行特征关注而获得云层空间分布信息,并引导云层去除;最后在构造的联合损失函数约束下训练模型,确保生成图像和真实影像在内容上的一致性。在遥感影像数据集RICE上的实验结果表明,本文提出的基于注意力机制和生成对抗网络的遥感影像云去除方法能够有效地保留影像边缘纹理细节,且达到了优异的定量指标和视觉效果。
High-resolution satellite remote sensing images are often compromised by cloud interference due to constraints such as imaging conditions and sensing equipment. This significantly degrades image quality and hinders intelligent interpretation. In recent years, deep learning has been extensively applied to cloud removal in remote sensing images, thanks to its powerful feature learning capabilities. This paper presents an attention-based generative adversarial network for cloud removal in remote sensing images, which aims to address the challenge of preserving textures. Initially, a Fourier residual block is designed to integrate both low-frequency and high-frequency of cloud images. Subsequently, attention mechanisms are employed from both channel and spatial to capture the spatial distribution information of clouds that guides the network in effectively removing clouds. Finally, the model is trained to minimize the joint loss function, ensuring the coherence of generated images with real images in terms of content. Experimental results derived from the RICE dataset substantiate that the proposed cloud removal method, which integrates attention mechanisms and generative adversarial networks, is capable of effectively eradicating clouds while preserving textures. The following conclusions can be drawn: (1) The proposed Fourier residual block plays a pivotal role in adeptly capturing the details of both low-frequency and high-frequency residual information present in cloud images. By merging these diverse features, the block bolsters the network’s ability to extract rich features; (2) The attention mechanisms utilized extract features from both spatial and channel dimensions. This enables the model to discern the significance of various features across different dimensions. Moreover, the cloud information obtained through these attention mechanisms allows the model to adaptively present the changes in cloud, thereby enhancing the model’s precision and efficiency in cloud removal; (3) Experimental results on the RICE dataset provide compelling evidence of the proposed method’s effectiveness in terms of cloud removal and texture preservation.