下载中心
优秀审稿专家
优秀论文
相关链接
首页 > , Vol. , Issue () : -
摘要
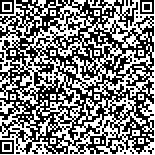
遥感图像中的阴影对图像解读和地物要素提取至关重要,但它们也可能成为干扰因素。当前,尽管深度学习在遥感图像阴影检测中取得了一定进展,但仍面临漏检、误检以及网络模型复杂等挑战。为此,本研究提出了一种融合Transformer与卷积神经网络(CNN)优势的双分支阴影检测网络。该方法采用双分支结构,从全局和局部两个层面深入挖掘信息,实现特征的有效整合,以更精确地捕捉阴影特征。此外,通过引入阴影预测模块和联合损失函数,进一步提升了阴影检测的准确性。在航空影像阴影数据集AISD(Aerial Imagery Shadow Dataset)上的实验结果表明,该方法显著提高了阴影检测的准确率,达到97.112%,并成功降低了误检率(BER降低了0.389),充分验证了其有效性。总之,本研究提出的双分支遥感图像阴影检测网络为减少小面积阴影的漏检与误检提供了新的有效手段,对遥感图像解译和地物要素提取具有重要意义。
(Objective) Shadows in remote sensing imagery play a crucial role in image interpretation and feature extraction but are also known to introduce significant challenges in image analysis. Traditional methods often struggle with complex shadow scenarios, leading to missed or false detections. This paper introduces a novel approach that enhances shadow detection accuracy and reliability in high-resolution remote sensing images. (Method) The proposed dual-branch network synergistically combines the strengths of Transformer and Convolutional Neural Networks (CNNs) to tackle the challenges of shadow detection. The network leverages a Transformer branch to capture global contextual relationships and a CNN branch to emphasize local textural details. This architecture is designed to exploit the complementary nature of global and local information, providing a comprehensive feature representation. This method also introduces a shadow prediction module that integrates these features for effective shadow segmentation. A joint loss function, comprising a primary loss and auxiliary losses, is utilized to refine learning and accelerate convergence, thereby enhancing the detection accuracy. (Result) The proposed method was rigorously tested on the Aerial Imagery Shadow Dataset (AISD), demonstrating substantial improvements in shadow detection metrics. It achieved a shadow detection accuracy of 97.112% and significantly reduced the false detection rate, with a balance error rate (BER) decrease of 0.389. These results not only validate the effectiveness of the dual-branch architecture but also showcase the advantages of integrating global and local features through our innovative network design. (Conclusion) The dual-branch network provides a robust solution to the perennial challenges of shadow detection in remote sensing imagery. By effectively minimizing missed and false detections, the network holds significant promise for enhancing the interpretability and utility of high-resolution satellite images in various applications, such as urban planning and environmental monitoring. The future work will focus on optimizing the network architecture and exploring its applicability to other complex imaging conditions.