下载中心
优秀审稿专家
优秀论文
相关链接
首页 > , Vol. , Issue () : -
摘要
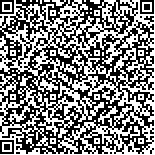
全天空卫星可见光反射率资料蕴含关键的云雨信息,具有重大的同化潜力。传统的可见光反射率资料同化观测算子基于数值方法求解辐射传输方程,计算效率偏低,尚不能满足资料同化的业务应用需求。鉴于此,本研究在Scheck(2021)神经网络观测算子的基础上,优化了神经网络参数与结构,构建了风云四号A星(FY-4A)先进静止轨道辐射成像仪(AGRI)可见光反射率资料同化的快速观测算子NNFO。NNFO的输入参数包括液相和冰相总云水路径(转换到对数空间)、冰相云水路径与总云水路径之比、液滴有效粒子半径、下垫面反照率、太阳天顶角、卫星天顶角、太阳与卫星的相对方位角,输出数据为对应条件下的天顶反射率。结果表明,NNFO的计算效率是传统观测算子RTTOV-DOM的15倍(串行)或6倍(并行),并且NNFO具有与RTTOV-DOM相当的模拟精度。此外,NNFO可以较好地重构FY-4A/AGRI可见光云图,反射率模拟结果的偏差和均方根误差分别为-0.016和0.143。因此,NNFO具有同化应用的潜力。
Satellite all-sky visible reflectance contain critical information on cloud and precipitation. Therefore, data assimilation (DA) of these data has great potential to improve the forecasting skills of numerical weather prediction (NWP) models. Conventional forward operators for the DA of visible reflectance are based on numerical methods to simulate the radiative transferring processes and suffer from high computational burden. Therefore, conventional forward operators cannot meet the needs for operational DA. Objective The study is designed to construct a fast and accurate forward operator for the DA of visible reflectance data provided by the Advanced Geostationary Radiation Imager (AGRI) onboard the Fengyun-4A satellite. The forward operator should be comparable to conventional forward operators in accuracy and overwhelms the latter in computational efficiency. Method A feed-forward neural network was utilized to construct the forward operator. The input parameters of the neural network-based forward operator (NNFO) include cloud water path converted into logarithmic space, the mixing ratio of ice cloud water path and the total cloud water path, the effective radius of cloud liquid droplets, underlying surface albedo, solar zenith angle, satellite zenith angle, and relative azimuth angle between the sun and the satellite. The output of NNFO is the top-of-atmosphere reflectance. A series of sensitivity studies were performed to find the optimal (or at least, the sub-optimal) neural network settings, which include 5 hidden layers, 57 nodes in each hidden layer, the “swish” activation function for the hidden layers, and 512 batch size. In addition, the neural network is trained with an adaptive learning rate depending on the training epoch and the loss for the validation dataset, which was defined by the root mean square error (RMSE). Result NNFO is compared with RTTOV-DOM, a typical forward operator based on discrete ordinate method to simulate the radiative transfer processes. The results indicate that NNFO is 15 or 6 times faster than RTTOV-DOM for serial or parallel modes. The mean difference, RMSE, and mean absolute error of the difference of reflectance simulated by RTTOV-DOM and NNFO (RTTOV-DOM minus NNFO) is 0.001, 0.048, 0.029, implying that the simulation accuracy between the two forward operators are comparable to each other. In addition, NNFO is validated by FY-4A/AGRI one-week reflectance observations. The results revealed that the probability density function of the simulation errors conforms to a Gaussian function, with the mean bias and standard deviation of -0.016 and 0.052, respectively. Conclusion NNFO is comparable to traditional forward operators in accuracy with a distinguished advantage in computational efficiency. Nevertheless, it is noteworthy that the current version of NNFO only supports the Ensemble Kalman Filter methods (including its variants). When it comes to the four-dimensional variational methods, NNFO should be developed by including its adjoint. In addition, NNFO could be further improved by including the aerosol effects. Improving NNFO in the aforementioned aspects and extending NNFO to DA applications are ongoing.