下载中心
优秀审稿专家
优秀论文
相关链接
首页 > , Vol. , Issue () : -
摘要
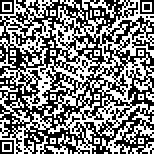
Transformer模型因其强大的全局特征建模和长距离依赖关系的表征能力现已广泛应用于遥感图像场景分类领域,但遥感场景图像存在空间结构复杂、目标尺度变化大等挑战,直接采用ViT(Vision Transformer)中固定尺寸的图像分块方式和深度特征表示不能有效刻画遥感场景图像的空间特征信息。针对上述问题,本文提出一种基于双阶段高阶Transformer(Two-stage High-order Vision Transformer, THViT)的遥感图像场景分类方法。该方法以LV-ViT-S网络为主干网,包含粗-细动态分类阶段,该阶段通过将遥感图像分割为较大尺度的图像块或根据类注意力机制和信息区域提取模块进行遥感图像再分块,完成易分类遥感场景图像和复杂遥感图像的场景分类。粗-细动态分类阶段可通过阈值调节。同时,为了提升深度特征的可判别性,THViT引入布朗协方差高阶特征表示,从统计学角度,有效捕获遥感场景图像的判别深度特征表示。另外,为了克服Transformer网络仅使用分类Tokens作为分类特征的局限性,本文将分类Tokens和高阶特征Tokens同时输入Softmax分类器,提升遥感图像场景分类性能,并验证了高阶特征Tokens对遥感图像场景分类的有效性。实验结果表明:与CFDNN、GLDBS、GAN、GCN、D-CapsNet、SCCov、ViT、Swin-T、LV-ViT-S和SCViT等相关算法对比,THViT在NWPU45(NWPU-RESISC45 Dataset)和AID(Aerial Image Dataset)数据集上均有较优异的性能表现。
TTransformer has been widely used in the field of remote sensing image scene classification because of its powerful global feature modeling and long-distance dependency representation capabilities. However, remote sensing scene images have challenges such as complex spatial structures and large changes in target scale. Directly adopting the fixed-size image block method and deep feature representation of ViT (Vision Transformer) cannot effectively depict the spatial feature information of remote sensing scene images. For alleviating the above problems, this paper proposes a remote sensing image scene classification method based on Two-stage High-order Vision Transformer (THViT). The method takes LV-ViT-S network as backbone, which includes a coarse-fine dynamic classification stage, then it divides remote sensing images into larger-scale image blocks or re-blocks them based on class-attention mechanism and information region extraction module for completing the classification of easily classified remote sensing scene images and complex remote sensing scene images, respectively. The coarse-fine dynamic classification stage can be adjusted by threshold. Simultaneously, for improving the discriminability of deep features, THViT introduces brownian covariance high-order feature representation, which effectively captures the discrimination depth feature representations of remote sensing scene images from a statistical perspective. Moreover, with the purposes of overcoming the limitation that transformer only utilizes classified tokens as classification features, this paper employs both classified tokens and high-order feature tokens into the softmax classifier for improving the performance of remote sensing image scene classification, and this style verifies the effectiveness of high-order feature tokens for remote sensing image scene classification. The experimental results illustrate that while compared with related algorithms such as CFDNN, GLDBS, GAN, GCN, D-CapsNet, SCCov, ViT and SCViT, THViT achieves better performance on the NWPU-RESISC45 dataset and AID dataset.