下载中心
优秀审稿专家
优秀论文
相关链接
首页 > , Vol. , Issue () : -
摘要
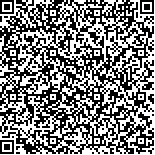
地物阴影的标注成本昂贵且难以全面覆盖高分辨率遥感影像蕴含的丰富信息,其训练样本的匮乏,严重限制了监督学习模型的性能。针对上述问题,本文提出了一种基于阴影方向先验的高分辨率遥感影像地物阴影检测方法,该方法探究了阴影方向先验对于遥感影像地物阴影高级语义特征表达的有效性,并基于阴影的方向性先验构建了遥感地物阴影检测辅助任务,实现自监督的遥感地物阴影检测方法。本文方法设计了一种方向变换无关性噪声处理机制和一种自监督阴影检测的数据增强策略,进一步提升深度神经网络对于地物阴影关键特征的学习能力。在AISD数据集上的实验结果表明,本文方法仅使用少量的标签即可显著提升地物阴影检测精度,并且地物阴影的边界更加平滑和规整,更接近于地面真实情况。
Objective. Due to the expensive and difficult of labeling of the shadows, the lack of training samples seriously limits the performance of supervised learning models for the shadow detection in high-resolution remote sensing images. In this paper, we propose a shadow detection method based on the shadow direction prior to learn the inherent information about shadow contained within unlabeled high-resolution remote sensing images. Methods. This paper proposes a high-resolution remote sensing image shadow detection method based on the prior knowledge of shadow direction. The method explores the effectiveness of shadow direction prior in representing advanced semantic features of object shadows in remote sensing images. It constructs an auxiliary task for remote sensing object shadow detection based on the directional prior of shadows, achieving a self-supervised shadow detection method. The proposed method designs a direction-invariant noise handling mechanism and a data augmentation strategy for self-supervised shadow detection to further enhance the deep neural network"s learning ability for key features of object shadows. Results. The effectiveness of this paper is validated on the AISD data set. Compared with the traditional Unet as a benchmark, the results show that the proposed method can learn the prior knowledge contained within the unlabeled high-resolution remote sensing images. By the prior knowledge, the proposed method can improve the accuracy of Unet, and achieve competitive efficiency and performance with small samples. Conclusion. The qualitative and quantitative results show that the method in this paper can improve the shadow detection accuracy with the shadow direction prior. The DTICT strategy can mitigate the interference of shadow-free samples and samples containing shadows with special directions during network training. A data augmentation strategy incorporating contrastive cropping and color transformations assist the network in obtaining more diverse training samples and enhancing the feature extraction capability of the pre-trained model for object shadows. The method achieves competitive object shadow detection accuracies with a small number of labeled samples.