下载中心
优秀审稿专家
优秀论文
相关链接
首页 > , Vol. , Issue () : -
摘要
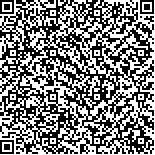
近年来,遥感智能解译技术快速发展,但大多为专用模型难以泛化到不同任务中,易造成资源浪费。基础模型是一种通用可泛化的解决方案,最近在遥感领域备受关注。尽管目前有大量工作已利用遥感单时相或多时相数据在感知识别和认知预测的部分任务上取得显著成果,但缺乏一个全面的综述给遥感基础模型提供系统的概述。因此本文首先从数据、方法和应用角度对现有遥感基础模型的研究进展进行总结,然后通过分析现状存在的局限提出新一代遥感通用预测基础模型的设想,最后针对亟需研究的方向进行探讨与实验,为研究人员提供遥感基础模型过去成果与未来可能性之间的桥梁。
In recent years, remote sensing intelligent interpretation technology has advanced rapidly, but most of them are task-oriented models. Therefore, it is difficult for them to generalize to different tasks, and it is easy to cause waste of resources. The foundation model is a straightforward approach that has recently attracted a lot of interest in remote sensing. Although many works have achieved remarkable results in some tasks of perception recognition and cognitive prediction by using remote sensing single-temporal or multi-temporal data, there is a lack of a comprehensive review to provide a systematic overview of the remote sensing foundation model. As a result, this paper begins by summarising the research developments of existing remote sensing foundation models from the perspectives of data, methods and applications. Then, after analyzing the current situation"s limits, we propose the idea of a new general predictive foundatation model. Finally, some very important research areas are highlighted, providing researchers with a link between past achievements and future possibilities of remote sensing foundation model. The existing remote sensing foundation models are categorised into three groups in this paper based on the data types used (single-temporal/multi-temporal) and the tasks involved (perceptual recognition/cognitive prediction): the foundation model of perceptual recognition based on single-temporal data, the foundation model of perceptual recognition based on multi-temporal data, and the foundation model of cognitive prediction based on multi-temporal data. According to the different self-supervised learning methods adopted, we divide the existing foundation models of perceptual recognition based on single-temporal data into those based on contrastive learning and those based on generative learning. According to the number of tasks, the foundation model of perceptual recognition based on multi-temporal data is divided into a single-task-oriented foundation model and a multi-task-oriented foundation model. According to different model architectures, the cognitive prediction foundation models based on multi-temporal data are divided into Transformer-based foundation models and graph network-based foundation models. In accordance with the aforementioned categorisation, we describe the current state of each type of remote sensing foundation models, and summarise their data, method, and application restrictions. Based on the summary and analysis of the existing remote sensing foundation models, we propose a new general predictive foundatation model assumption. The information pipeline for multi-domain/temporal data input and multi-time/spatial scale task output can be opened up by extracting stable and generalised time-series hyper-pixel features in order to achieve accurate cognitive prediction of the future state. In terms of data, it specifically includes tens of millions of multi-platform, multi-type, multi-modal, and multi-temporal data. In terms of methods: By combining the benefits of the Transformer model and the graph network, a new foundation model architecture is created, which increases the model"s capacity and enhances generalisation while also having the ability to predict multi-target interactions in large remote sensing scenes over the long term. In terms of application: the general predictive foundation model can be applied to diverse cognitive prediction tasks with multiple spatial scales and multiple time scales. Under this assumption, we propose four exploratory directions: multi-domain time-series data representation, stable feature extraction, object-environment interaction modeling, and multi-task interaction reasoning, for the reference of researchers working on remote sensing foundation models. In general, foundation models with generalization ability are crucial for the further development of remote sensing intelligent interpretation. We provide researchers with an overview of the latest advances in this field by collating the current state of research on remote sensing foundation models. On this basis, by analyzing the limitations of current remote sensing foundation models in terms of data, methods, and applications, this paper puts forward a new general predictive foundatation model assumption, and further clarifies four exploratory directions that urgently need breakthroughs under this idea. The follow-up work will make specific, significant technological breakthroughs in multi-domain time-series data representation, stable feature extraction, object-environment interaction modeling, and multi-task interaction reasoning. At the same time, we continue to explore a more general remote sensing foundatation model, integrating perception recognition and cognitive prediction into one architecture.