下载中心
优秀审稿专家
优秀论文
相关链接
首页 > , Vol. , Issue () : -
摘要
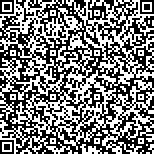
DOI:
收稿日期:
2023-07-13
修改日期:
2024-02-10
近年来突发性地表异常ESA(Earth Surface Anomalies)事件频发且呈上升趋势,给人类的生命、财产安全带来了巨大威胁,如何及时准确地发现地表异常事件对后续救援与灾害响应具有重要意义。一些研究人员利用卫星影像开展大尺度地表异常发现与监测,并开发、运用了先进的深度学习方法。然而,由于标签数据不足和卫星影像中几何、语义信息十分复杂,现阶段的地表异常检测方法的表现往往不能达到很好的效果。针对上述问题,我们提出了一个多层级几何-语义融合的图神经网络GNN(Graph Neural Network)框架,以实现高精度地表异常快速发现。具体而言,提出的方法首先利用两个不同的分支基于输入的卫星影像提取不同层级的地理实体并构建图表示,并通过分配矩阵实现图的联合表达。此后,我们构建了分层图注意力网络GAT(Graph Attention Network),基于图节点信息传递、聚合与注意力机制从图中进一步挖掘卫星影像中复杂的几何、语义信息,用于准确地检测地表异常。考虑到现有大规模多类地表异常检测基准数据集的缺乏,我们基于现有公开可分发数据集构建了ESAD数据集,用于基于单张卫星影像开展地表异常检测任务。大量实验表明,提出的方法在地表异常检测任务中取得了优异的性能,在准确率、召回率与推理时间方面优于许多基线方法,可精确、有效地开展地表异常检测任务。
The increasing occurrence of earth surface anomalies (ESA) highlights the importance of timely and accurate detection of such events. Therefore, researchers have utilized satellite imagery for large-scale detection and developed advanced deep learning methods. However, the performance is hindered by inadequate labeled data and the complexity of semantic information in satellite imagery. In this paper, we aim to address the aforementioned problems and improve the performance in earth surface anomalies detection. This paper firstly reviews and summarizes the development of Earth’s surface anomalies detection, and the problems that hinder the performance of existed methods. Then we propose a hierarchical geometry-to-semantic fusion graph neural network (GNN) framework, which utilizes single image for the detection of Earth’s surface anomalies, thereby reducing the demand for data and decreasing the time required for preprocessing and inference. Specifically, our method employs two branches to extract geoentities and construct graphs at different levels. And then a hierarchical graph attention network (GAT) is utilized to update the node features and extract graph embeddings for each level. An attention-based feature fusion module then combines them to yield the graph-level feature vector of the input image, which is finally processed through a Multi-Layer Perceptron (MLP) for ESA detection. Considering that the existing ESA datasets mainly focus on single-class detection or post-hoc analysis, which is insufficient for our research needs, we propose ESAD, a composite dataset to bridge the gap of large-scale multi-class datasets. Specifically, ESAD is composed of three publicly datasets: xBD, Multi3Net and Sichuan Landslide and Debrisflow. Extensive experiments demonstrate that the proposed method is effective and accurate for detecting ESA, outperforming many baseline methods. Our method strikes a good balance between accuracy and efficiency, making it more suitable for ESA detection while saving valuable time and resources for downstream tasks. In conclusion, we propose a hierarchical geometry-to-semantic fusion GNN framework for ESA detection. It leverages GNN to learn high-order semantic information from the satellite imagery. To deal with the lack of benchmark datasets, we created the ESAD dataset based on existing related datasets. We have done many extensive experiments to demonstrate that our method achieves a good balance between accuracy and efficiency, which is more suitable for ESA detection with high timeliness requirements. In future work, we will further explore more models for better performance and extend our method to on-orbit real-time ESA detection task.