下载中心
优秀审稿专家
优秀论文
相关链接
首页 > , Vol. , Issue () : -
摘要
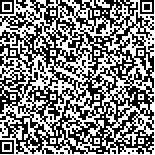
遥感有向目标检测是计算机视觉领域内一项具有挑战性的任务,传统的水平框表示法无法精确定位尺度各异、方向任意且密集排列的遥感目标。目前广泛采用的五参数有向框表示法,由于方向角的周期性和边的交换性问题,增加了模型训练的复杂度。为了解决上述问题,本文提出了一种基于椭圆方程的遥感有向目标检测模型(Elliptical Equation-based Remote Sensing Oriented Object Detection Network,EllipticNet)。首先,EllipticNet将方向角的预测问题解耦为两个子问题:定量角度回归和旋转方向分类,从而克服五参数有向框表示法的边界不连续性问题;结合椭圆的长短轴以及中心点预测,实现遥感有向目标的精确表示。其次,本文设计了一种椭圆约束的损失函数,通过增强椭圆参数之间的内在几何关系,提高EllipticNet训练的鲁棒性。此外,本文还提出了一种逐层空洞空间卷积池化金字塔模块,显著提升EllipticNet对多尺度特征的表征能力。最后,在DOTA、HRSC2016和UCAS_AOD三个常用的公开遥感数据集上的对比实验表明,本文方法在性能和效率方面均具有竞争力,表明本文方法在遥感有向目标检测中具有一定的实用价值。
Remote sensing directed target detection is a challenging task in the field of computer vision, and the traditional horizontal frame representation cannot accurately locate remote sensing targets with different scales and arbitrary and densely arranged orientations. The five-parameter directed box representation, which is widely used nowadays, increases the complexity of model training due to the periodicity of direction angles and the problem of edge exchange. In order to solve the above problems, an Elliptical Equation-based Remote Sensing Oriented Object Detection Network (EllipticNet) is proposed in this paper. Firstly, EllipticNet decouples the prediction problem of orientation angle into two sub-problems: quantitative angular regression and rotational direction classification, so as to overcome the boundary discontinuity problem of the five-parameter oriented frame representation; and combines the long and short axes of the ellipse as well as the prediction of the centroid point to realize the accurate representation of remote sensing oriented targets. Secondly, this paper designs an ellipse-constrained loss function to improve the robustness of EllipticNet training by enhancing the intrinsic geometric relationship between elliptic parameters. In addition, this paper proposes a layer-by-layer null-space convolutional pooling pyramid module, which significantly improves EllipticNet"s ability to characterize multi-scale features. Finally, comparison experiments on three commonly used publicly available remote sensing datasets, DOTA, HRSC2016 and UCAS_AOD, show that this paper"s method is competitive in terms of performance and efficiency, indicating that this paper"s method has some practical value in remote sensing directed target detection.