下载中心
优秀审稿专家
优秀论文
相关链接
首页 > , Vol. , Issue () : -
摘要
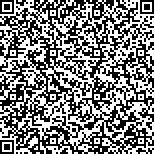
超分辨率重建技术在卫星遥感图像信息智能处理领域中有重要的应用。现有面向遥感图像超分辨率重建的深度学习方法大多只能处理一种比例因子的超分辨率重建任务,在多尺度层面上缺少泛化性,难以满足真实遥感图像多倍率连续放大的超分辨率重建任务需求。为解决遥感图像超分辨率重建过程中的多尺度放大问题,本文采用元学习的方法,在构建单一自适应模型的基础上实现对遥感图像的任意尺度超分辨率重建,提升遥感图像的空间分辨率,利用密集残差网络和通道注意力机制重建遥感图像中地物纹理、目标边缘等丰富细节信息,在真实遥感数据上的定量和定性实验结果证明了本文方法的有效性。
Super-resolution reconstruction technology plays an important role in the intelligent processing of satellite remote-sensing image. Existing deep learning methods for remote-sensing image super-resolution reconstruction can only handle super-resolution tasks with a single scale factor, lacking generalization at the multi-scale level and unable to meet the requirements of real remote-sensing image super-resolution reconstruction for continuous zooming at multiple magnification levels. To address the problem of arbitrary-scale super-resolution reconstruction in remote sensing images, this paper proposes a super-resolution reconstruction method called Meta-RDCAN, which utilizes meta-learning and residual dense channel attention network. The proposed method employs a meta upscale module that incorporates three functions: weight prediction, location projection, and feature mapping, to achieve arbitrary-scale super-resolution reconstruction. Additionally, from the perspective of extracting detailed information of local land objects in remote-sensing images, a residual dense channel attention feature extraction network is designed to restore the fine details of remote-sensing images.