下载中心
优秀审稿专家
优秀论文
相关链接
首页 > , Vol. , Issue () : -
摘要
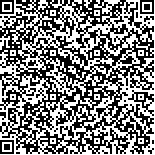
光学遥感是航天侦察和地质勘测中的常用技术,拍摄得到的可见光图像能够提供非常丰富的信息,在情报侦察、目标监视、态势预判等方面都具有重要应用。近年来以轮船、飞机等物体检测为代表的遥感图像目标感知取得了显著进展,但对于目标尺度变化大,目标数量多而小的遥感图像目标感知场景中还存在巨大技术挑战。现有工作主要关注于改进边界框的表示,而单目标的检测模式无法充分利用其周围物体或同类物体的空间关联信息。为了解决现有遥感图像目标检测算法不同目标独立检测的内在低效性,本文提出了一种新的检测框架,即群目标检测,通过检测群目标的状态信息来缓解单一目标感知信息不足、可靠性差等问题,进而得到更为可靠的多目标检测结果。此外,本文提出了一种群目标自动化标注方案,在公开数据集上对原有标签进行分析,无需任何手动标注,通过数量和位置分布自适应地调整聚类数量,利用聚类算法将原始图片分为几个集群,并给出集群标注信息。基于群目标标注,本文提出了一个群目标检测算法,在检测群目标的同时,利用群目标的空间约束从而提升单一目标检测结果。在最热门的大型遥感目标检测数据集DOTA上,该方法取得了最佳的性能。
Optical remote sensing is a widely-used technology in aerospace reconnaissance and geological exploration. Visible light images captured by this technology provide a wealth of information and have important applications in intelligence gathering, object monitoring, and situational forecasting. Recent years have seen significant progress in remote sensing image object perception, with ship and airplane detection being representative examples. However, there remain significant technical challenges in remote sensing image object perception scenarios with large object scale variations and numerous, small objects. Existing work has mainly focused on improving boundary box representations, but single-object detection models fail to fully exploit spatial correlation information from surrounding or similar objects. To address the inherent inefficiency of existing remote sensing image object detection algorithms that detect different objects independently, this paper proposes a novel detection framework called group object detection. By detecting the state information of group objects, our framework alleviates problems such as insufficient perception information and poor reliability of single-object perception, leading to more reliable multi-object detection results. Additionally, this paper presents an automated annotation scheme for group objects, which analyzes original labels on public datasets and adaptively adjusts the clustering quantity based on the number and position distribution. The scheme utilizes clustering algorithms to divide original images into several clusters and provide cluster labeling information. Based on group object annotation, this paper proposes a group object detection algorithm that improves single-object detection results by utilizing spatial constraints of group objects while detecting them. The proposed method achieved state-of-the-art performance on the popular large-scale remote sensing object detection dataset, DOTA.