下载中心
优秀审稿专家
优秀论文
相关链接
首页 > , Vol. , Issue () : -
摘要
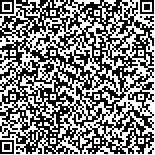
目的 基于遥感图像的目标细粒度分类深度神经网络已经在军事和民用领域得到广泛应用,网络决策的可解释性研究是当前细粒度分类深度学习算法进一步提高决策可信度的关键问题。方法 本文基于博弈竞争理论对遥感图像目标细粒度分类任务进行建模,分析了IG、SmoothGrad、Grad-CAM等可解释性方法在遥感图像目标细粒度分类网络上的适用性,提出了一种尺度自适应的目标细粒度分类本质特征可解释性分析方法Hybrid-Grid,使用像素级与局部特征关系融合算法提高对支撑网络决策的目标本质特征的精确描述能力。结果 实验结果表明,本文提出的Hybrid-Grid对目标细粒度分类网络的解释效果在ADCC量化评估指标上达到78.87,相较Score-CAM有大幅提升。与SmoothGrad、Grad-CAM的解释结果相对比,本文方法在删除及精度损失实验上表现最好,使EFM-Net的Top-1准确率、Top-5准确率、F1得分分别损失了16.92%、1.61%、17.21%,证明Hybrid-Grid准确解释了对细粒度分类网络决策贡献最大的目标本质特征。结论 本文提出的可解释性分析方法能够更准确地揭示当前目标细粒度分类网络的决策特征依据。
Objective At present, the deep neural network for targets fine-grained classification based on remote sensing images has been widely applied in military and civilian fields. The "black box" problem of deep learning network models still makes it difficult for people to understand the decision-making basis of the network in fine-grained classification tasks. This not only limits the possibility of deep neural networks optimizing and improving through feedback guidance, but also makes them unable to be fully trusted by humans and applied in important fields such as military and medical fields. Thus, how to carry out explainability research on its internal decision-making mechanism is the key problem for the current fine-grained algorithm to further improve the credibility of decision-making basis. Method This article summarized the commonly used explainable methods in existing fine-grained classification networks for remote sensing image targets. On this basis, a mathematical model for the problem of target fine-grained classification is established. It also models the fine-grained classification task of remote sensing image targets based on game competition theory, analyzes the applicability of explainable methods such as IG, SmoothGrad, and Grad CAM on the fine-grained classification network of remote sensing image targets, and proposes a scale adaptive method for analyzing the explainability of essential features of fine-grained classification of targets, that is, Hybrid Grid. The fusion algorithm of pixel level and local feature relationships is used to improve the accurate description ability of essential features of targets that support network decision-making. Result The typical explaining methods involved in this article were evaluated using indicators such as Average Drop, Coherence, Complexity, ADCC, and Deletion and precision loss, confirming the accuracy of the proposed method. The experimental results show that the Hybrid-Grid proposed in this paper achieves a score of 78.87 on the quantitative evaluation index of ADCC for target fine-grained classification networks, which is significantly improved compared to Score-CAM. Compared with the explanation results of SmoothGrad and Grad-CAM, our method performed the best in deletion and accuracy loss experiments, resulting in a loss of 16.92%, 1.61%, and 17.21% in the Top-1 accuracy, Top-5 accuracy, and F1 score of EFM Net, respectively. This proves that the Hybrid Grid accurately explains the essential feature of the target that contributes the most to fine-grained classification network decision-making. Conclusion Based on several typical fine-grained classification networks, the feasibility and universality of the explaining method proposed in this paper have been confirmed. The explaining method proposed in this article can more accurately reveal the decision feature basis of the current target fine-grained classification network. Future work will further enhance the visualization and interpretation accuracy of the essential features of the target, assisting in improving the classification performance and decision-making credibility of fine-grained classification networks.