下载中心
优秀审稿专家
优秀论文
相关链接
首页 > , Vol. , Issue () : -
摘要
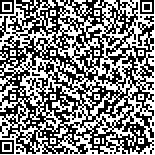
以残差网络为基础,结合高分辨率遥感影像的特点,提出一种全新的端到端变化检测网络--混合空间金字塔池化网络(Hybrid spatial pyramid pooling network,HSPPNet)。HSPPNet首先将空洞卷积和注意力机制引导卷积并行集成,构建一种混合空间金字塔池化模块,以便有效提取高分辨率遥感影像中不同形状尺度的变化对象。然后,通过定义一种截断-补偿加权交叉熵函数和一种类别级IoU函数并将二者集成,得到一种全新的自适应平衡损失函数,来降低变化类与未变化类严重不均衡问题对变化检测的影响。最后设计一种简单有效的输入模块,通过综合考虑两期遥感影像及其差异图来增强变化信息。通过以上三点,HSPPNet增强了深度学习变化检测的性能。两组常用公开变化检测数据集上的实验结果表明HSPPNet可行、有效。
Objective: Change detection (CD) is the process to identify the land cover changes of interest, by analyzing bi-temporal remote sensing images acquired in the same geographical area at different times. As a hot research topic in remote sensing, CD plays an important role in many practical applications, such as urban research, environmental monitoring, and disaster assessment. With the rapid development of deep learning, its applications have expanded to the remote sensing CD task, leading to the development of numerous deep learning-based CD methods. Although the existing deep learning-based CD methods have their own advantages and can implement the CD task effectively, their applicability and robustness need to be further analyzed. How to design an appropriate network model to obtain more reliable and accurate CD results is still an open problem. Currently, there are still two main challenges for CD: 1) The changed objects existing in remote sensing images have various scales and shapes, and 2) the proportions of the changed and unchanged classes are often significantly unbalanced, i.e., the number of the changed pixels are usually much less than that of the unchanged pixels. Method: To deal with these two challenges, this paper proposes a novel end-to-end CD network for high resolution remote sensing images based on the residual network, i.e., hybrid spatial pyramid pooling network (HSPPNet). First, a hybrid spatial pyramid pooling (HSPP) module is built by integrating atrous convolutions and attention-mechanism-guided convolutions in parallel, in order to effectively extract the changed objects with different shapes and scales from high-resolution remote sensing images. Then, an adaptive balancing loss function is presented to alleviate the impacts of the serious imbalance between changed and unchanged classes on CD. The loss function is constructed by defining a truncation-compensation weighting cross entropy function and a class-level IoU function and integrating them. Finally, a simple but effective input module that considers both the bi-temporal remote sensing images and their difference image is designed to enhance the change information. Owing to the above three points, the proposed HSPPNet method enhances the performance of deep learning CD. Result: Experiments were carried out with two public CD datasets, namely the CDD and Google datasets, in order to evaluate the performance of the proposed HSPPNet method. Six state-of-the-art deep learning methods were used as the comparative methods. Quantitative analysis of CD results was done on four evaluation metrics, namely precision, recall, overall accuracy, and F1-score. For the CDD and Google datasets, the overall accuracy/F1-score values of the proposed HSPPNet method are 0.9943/0.9758 and 0.9399/0.9076, respectively, which are superior to the six comparative methods. Conclusion: The experimental results on two public CD datasets demonstrate that the proposed HSPPNet is effective and feasible. In addition, the results of ablation experiments show that all of the HSPP module, the adaptive balancing loss function, and the input module proposed in this work can enhance the CD performance effectively.