下载中心
优秀审稿专家
优秀论文
相关链接
首页 > , Vol. , Issue () : -
摘要
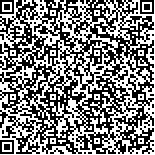
撞击坑作为月球表面上最典型和最常见的地貌单元,它们的形态特征和空间分布记录着月球的演化历史、气候形成以及月球表面年代。由于撞击坑边缘模糊、撞击坑嵌套等现象,导致撞击坑(尤其是小尺度撞击坑)自动识别仍存在识别精度不高、大尺度撞击坑中的小尺度撞击坑难以不易识别等问题。因此,针对小尺度撞击坑数量众多且不易识别,以及嵌套撞击坑识别等问题,本文提出了一种基于迁移学习策略的无锚深度卷积神经网络月表小尺度撞击坑自动识别方法,来自动定位和识别撞击坑。该方法在堆叠沙漏网络生成的高分辨率特征图上直接回归出撞击坑的中心及其大小,并且无需使用非极大值抑制等后处理操作,实现了不同类型撞击坑的自动识别。同时,采用迁移学习的思想进行训练,为模型训练赋予一定的优化参数,使其并非从头开始训练,从而使模型具备更高的可靠性和稳健性。文中选择虹湾和风暴洋月表地区的影像进行实验验证,并与其现有人工标注的Robbins撞击坑数据库进行定量与定性评估,结果表明本文设计的自动识别模型能有效地实现撞击坑提取,且一定程度上解决了嵌套撞击坑中小尺度撞击坑的识别。
Objective: Impact crater are the most typical and common geomorphic units on the lunar surface. Their morphological WUcharacteristics and spatial distribution record the evolution history, climate formation and surface age of the moon. Due to the impact crater’ fuzzy edges and nested impact craters, the automatic recognition of impact craters (especially small-scale one) still faces problems such as low accuracy, and difficulty in identifying small-scale within large-scale impact crater. Therefore, to address the mentioned issues, this paper proposes an automatic anchor-free convolutional neural network method based on transfer learning strategy to automatically locate and identify small-scale lunar impact craters. Methodology: Instead of using post-processing operations such as non-maximum suppression, the proposed method directly locates the centers of impact craters and regresses their sizes on the high-resolution feature map generated by the stacked hourglass network, thus realizing the automatic recognition of different types of impact craters. At the same time, the idea of transfer learning is used for training, not from scratch, so that the model has higher reliability and robustness. Results: This paper selects the Orthophoto image captured by LRO WAC camera on the Lunar Reconnaissance Orbiter and the Robbins impact crater database in the Sinus Iridum and Oceanus Procellarum region to verify the reliability and robustness of the developed method. The method in this paper achieves a recall rate of up to 74.71% and an accuracy of up to 75.97%. Compared with other existing methods, it shows remarkable advantages in recognition accuracy, and has a high adaptability in extracting and identifying impact crater in different areas of the lunar surface. When comparing the performance and accuracy by drawing roc curves of different models, it can be concluded that the idea of Transfer learning can effectively help the models converge better and improve the performance of model classification. Conclusions: The automatic recognition model in this paper can effectively achieve the extraction of impact craters and can to a certain extent solve the identification of small and medium-sized impact craters within nested impact craters. The key insight behind the developed method is to improve the model recognition accuracy based on transfer learning. Meanwhile, it does not need the non-maximum suppression operation, which would effectively realize the identification of small and medium-sized ones within nested impact craters. Although the developed method shows a superior recognition performance for the lunar impact craters, there are still the following shortcomings. The generalization of the model still needs to be further improved. Therefore, in the future work, we will also make full use of the similarity of impact crater in different regions to reduce the rate of missed detection of impact crater on the lunar surface.