下载中心
优秀审稿专家
优秀论文
相关链接
首页 > , Vol. , Issue () : -
摘要
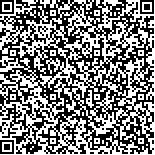
地块作为农业耕作的最小单元,对其精准识别是国土资源监测、耕地利用监测的需要。现有的方法多使用手工勾绘的方式获取,耗时费力,成本高昂,并且无法实现实时、近实时更新。本文设计了一种基于空间注意力机制与多任务学习的地块分割模型—Field-Net。模型基于UNet架构,增加了空间注意力机制,并采用多任务学习的策略,在语义分割的基础上增加了边界、像素到地块边界的距离等任务。在山东省东营市利津县对模型的性能进行了测试,结果发现耕地地块识别的交并比达到了87.05%,总体精度为92.23%。Field-Net模型的性能优于几种高性能的深度学习框架,交并比较Link-Net模型高出0.26%,较DeepLab v3+高出7.59%。在空间泛化性能测试中,Field-Net模型的平均交并比Link-Net模型高出3.51%,空间泛化性能明显提升。通过消融试验发现,使用空间注意力机制的Field-Net较ResUNet模型F1-Score提高了1.01%,交并比提高了1.6%;多任务学习策略使得Field-Net模型的F1-Score提高了0.18%,交并比提高了0.21%;将模型权重特征进行可视化后发现空间注意力机制模块和多任务学习策略可以使模型学习到的特征更加聚集于地块边界和地块内部,使学习到的特征更具代表性。总体而言,Field-Net模型可以支撑地块级别国土资源和耕地非农化、非粮化利用监测,从而提高监测的效率和时效性。
Objective: Accurate identification of agricultural fields, the smallest units of agricultural farming, is crucial for monitoring land resources and arable land utilization. Manual mapping methods are time-consuming and expensive, incapable of real-time or near-real-time updates. To enhance agricultural field delineation efficiency, we introduce Field-Net, a field segmentation model leveraging spatial attention mechanisms and multi-task learning in this research. Method: Field-Net is based on the UNet architecture, integrating spatial attention mechanisms and a multi-task learning approach. In addition to the segmentation task, we incorporate boundary identification and distance to the field boundary as two additional tasks, enabling the model to learn more representative features related to fields. The model"s performance was evaluated using GF-1 and ZY-3 satellite images with a spatial resolution of 2 meters in Lijin County, Dongying City, Shandong Province. We labeled 3,480 tiles measuring 256x256 pixels in the YanWo district, with 3,000 used for training, 360 for validation and 120 for spatial generalisation performance test. Results: Initially, we analyzed the loss weights for the three tasks—mask, boundary, and pixel-to-boundary distance—in multi-task learning using a gradient test. We found that for multi-task learning, the loss weights should prioritize the mask segmentation task as the primary task and the others as secondary. Across the entire test set, Field-Net achieved an overall accuracy of 92.23% and an IOU of 87.05%. We compared Field-Net with four state-of-the-art architectures: DeepLabv3+, HRNet, LinkNet, and D-LinkNet. Field-Net outperformed them all in semantic segmentation tasks, with an IOU 0.26% higher than Link-Net, the most accurate among the four selected models, and 7.59% higher than DeepLab v3+. In the spatial generalisation performance test, the average IOU of Field-Net model is 3.51% higher than that of Link-Net model, and the spatial generalisation performance is significantly improved. Ablation tests demonstrated that the spatial attention mechanism and multi-task learning strategy improved F1-Score by 1.01% and IOU by 1.6% compared to the ResUNet model. The multi-task learning strategy led to a 0.18% F1-Score improvement for Field-Net and a 0.21% improvement in IOU. Conclusion: While challenges remain in identifying contiguous fields due to unclear boundaries, future enhancements could incorporate multi-temporal and higher-resolution remote sensing images to improve field feature discrimination. Feature visualization analysis revealed that the spatial attention mechanism and multi-task learning strategy enabled the model to learn clustered features at field boundaries and within plots, enhancing feature representativeness. Overall, the Field-Net model supports field-level monitoring of cropland use, including non-agricultural applications, such as grain production, enhancing the efficiency and timeliness of land resource monitoring. In the process of generating the field dataset in China, the complex and fragmented cropland bring considerable challenges to this task. In the future, the problem of lack of samples for model training can be solved by accumulating field segmentation datasets from different regions by borrowing the paradigm of Image-Net, while a more general model for channels, regions, and sensors should be constructed subsequently. In the future, with the arrival of the "large model" era of deep learning, for the task of parcel segmentation, it is also necessary to construct a model to segment every field from the perspective of both the model and the dataset.