下载中心
优秀审稿专家
优秀论文
相关链接
首页 > , Vol. , Issue () : -
摘要
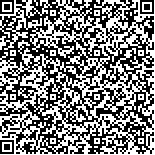
目前,许多深度学习点云分类方法通过增加点云特征聚合模块,增强点云特征的表达能力。但该类方法往往会带来训练参数增加以及模型过拟合的问题。针对该问题,本文提出了一个整合集成预测约束与错误预测熵最大化的深度学习方法用于移动激光扫描(Mobile Laser Scanning, MLS)点云分类。方法通过集成预测约束分支以及错误预测熵最大化分支可以在不增加训练参数的情况下,增强基线网络的点云特征表达,提高模型泛化能力。其中集成预测约束分支首先通过记录点云在训练过程中的预测值,生成集成预测值,然后采用一致性约束增强模型的点云特征表达。错误预测熵最大化方法鼓励模型对错误预测点进行熵值最大化,增加该点的不确定性,提高模型的泛化能力。所提方法在多个公开MLS点云数据集上进行验证,结果表明所提方法可以在不增加训练参数的情况下,提高基线方法的分类性能。与其他方法相比,所提方法在Toronto3D、WHU-MLS、Paris数据集上获得了最优的平均交并比(83.68%、44.19%、65.85%),表明了方法的有效性。
Abstract Objective Mobile Laser Scanning (MLS) systems are widely used in various industries due to their ability to rapidly acquire high-precision and high-density 3D laser point cloud data, especially in the acquisition of spatial information of urban scenes. Since MLS point clouds in urban areas with complex scenes, large data volume and uneven spatial distribution, there are significant challenges to accurately classify the large-scale point clouds. Currently, many deep learning large-scale point cloud classification methods enhance the representation of point cloud features by adding the point cloud feature aggregation module. However, this method often brings the problem of increasing training parameters and model over-fitting. Abstract Objective Mobile Laser Scanning (MLS) systems are widely used in various fields, owing to their ability for rapidly acquiring high-precision and high-density 3D point cloud data, particularly in the acquisition of urban spatial information. Since urban MLS point clouds exhibit complex scenes, large data volumes and uneven spatial distribution, accurate classification of large-scale urban point clouds presents significant challenges. Currently, many deep learning point cloud classification methods enhance feature representation of point cloud by adding the feature aggregation module. Nonetheless, this approach frequently results in increased training parameters and model over-fitting. Methods We propose an MLS point cloud classification method integrating resemble prediction constraints and error prediction entropy maximization. The proposed method is able to enhance the point cloud feature representation of the baseline network and improve the generalization ability of the model without increasing the training parameters. Our method consists of three main components: a basic supervision branch, an ensemble prediction constraint branch, and an error prediction entropy maximization branch. Specifically, we first employ RandLA-Net as the backbone network to obtain point cloud classification features. Then a basic supervised branch calculates the weighted cross-entropy loss based on the true labels, predicted probability distributions and category weights to provide the basic fully supervised signal for model training. For the ensemble prediction constraint branch, we first generate the ensemble predictions by recording the predicted values during the point cloud training process. Since the input to RandLA-Net is a random sub-point cloud, the ensemble predictions can be integrated not only for predictions at different stages, but also at different relative positions. Thus, the ensemble prediction is more robust to the current prediction, and then we apply the consistency constraint to minimize the difference between the two predictions to improve the point cloud feature representation. Finally, we design the error prediction entropy maximization branch to maximize the entropy of error prediction point sets, increasing their confusion to reduce the model over-fitting. Results and conclusion The public MLS point cloud dataset Toronto3D is chosen as the primary dataset to validate the performance of the proposed method. The qualitative result (Fig. 5) and quantitative result (Table 1) on the Toronto3D dataset show that the proposed method can correctly classify most points. To verify the validity of the method, we compare the accuracy of the proposed method with other popular methods. The comparison results (Table 1 and 2) show that the proposed method obtains the best OA and mIoU, in which the OA and mIoU can achieve 97.71% and 83.68%, respectively. To verify the effectiveness for each branch of the proposed method, a series of ablation experiments were carried out. The results (Table 3 and Fig. 7) show that each branch can effectively improve the model classification performance. The complexity analysis (Table 4) indicate that the proposed method can improve the accuracy of the baseline method without increasing the model parameters. Finally, the experimental results (Table 5) on other public MLS datasets (WHU-MLS and Paris datasets) show that the proposed method can obtain competitive results on multiple datasets. Results and conclusion The public MLS point cloud dataset Toronto3D is chosen as the primary dataset to validate the performance of the proposed method. The qualitative result (Fig. 5) and quantitative result (Table 1) on the Toronto3D dataset show that the proposed method can correctly classify most points. To verify the validity of the method, we compare the accuracy of the proposed method with other popular methods. The comparison results (Table 1 and 2) show that the proposed method obtains the best OA and mIoU, in which the OA and mIoU can achieve 97.71% and 83.68%, respectively. To verify the effectiveness for each branch of the proposed method, a series of ablation experiments were carried out. The results (Table 3 and Fig. 7) show that each branch can effectively improve the model classification performance. Finally, the complexity analysis (Table 4) and the experimental results (Table 5) on other MLS datasets (WHU-MLS and Paris datasets) indicate that the proposed method can improve the accuracy of the baseline method without increasing the model parameters.