下载中心
优秀审稿专家
优秀论文
相关链接
首页 > , Vol. , Issue () : -
摘要
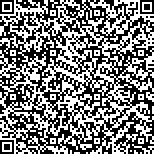
在光学遥感图像的各种应用中,目标检测历来受到研究人员的重视,并且在军事和民用方面都有很广泛的应用前景。本文综述了基于深度学习的光学遥感图像目标检测算法研究进展。首先,详细分析了光学遥感图像中的目标特点;然后,简要回顾了光学遥感图像目标检测算法的发展历程;其次,阐明了基于深度学习的光学遥感图像目标检测流程;接着,以深度学习为出发点,概述了经典的目标检测框架,并根据光学遥感图像目标的特点,分别针对尺度多样性、方向多样性、形状多样性、小尺寸、特征相似性、背景复杂性、分布密集性、弱特征的光学遥感图像目标检测问题,对各种改进算法进行了系统性的总结,此外,归纳了针对光学遥感图像目标检测的非全监督学习算法;随后,介绍了常用的开源光学遥感图像数据集和目标检测的性能评估指标;最后,指出了现阶段在光学遥感图像目标检测领域仍然面临的主要挑战和下一步的发展方向。
Abstract: In all kinds of applications of optical remote sensing image, object detection has always been paid more attention by researchers. It has a very wide application prospect in military and civilian aspects. This paper reviews the research progress of object detection algorithms in optical remote sensing images based on deep learning. The characteristics of remote sensing objects are different from those of conventional objects. Firstly, remote sensing equipment has a long imaging distance, so it can cover a large range. There may be objects with large scale and shape changes in the images. Secondly, in remote sensing images, the background tends to occupy a large area. This leads to the object often submerged in the complex background, which makes it difficult for the detectors to distinguish the objects effectively. Thirdly, in remote sensing images, the objects are not only reflected in the aspects of small size and changeable direction. Sometimes the remote sensing objects are often densely distributed, which brings some challenges to the detection of optical remote sensing objects. Firstly, this paper introduces the development process of optical remote sensing object detection algorithms from template matching, prior knowledge, and machine learning to deep learning. Then, the process of optical remote sensing object detection based on deep learning is introduced in detail, including data preprocessing, feature extraction, detection and post-processing. Thirdly, the classical deep learning-based object detection algorithms, including the one-stage algorithms represented by YOLO and SSD as well as the two-stage algorithm represented by Faster RCNN are summarized. Fourthly, according to the characteristics of optical remote sensing image object s, various improved algorithms are systematically summarized for optical remote sensing image object detection problems of scale diversity, direction diversity, shape diversity, small size, feature similarity, background complexity, distribution density and weak features. Fifthly, non-strong supervised learning based optical remote sensing image object detection methods and other advanced algorithms, such as transformer based algorithms, transfer learning based algorithms, knowledge graph based algorithms and prior knowledge based algorithms are summarized. In addition, the open source optical remote sensing image datasets and the performance of object detection evaluation indexes are introduced. At present, mAP of advanced algorithms in NWPU-VHR10 dataset can reach more than 90%. For DOTA dataset, mAP of each advanced algorithm has decreased significantly, and the advanced algorithms proposed in recent years still continue to improve the performance of this dataset. Multi-scale fusion with feature pyramid network has become the mainstream method of advanced algorithms, which can detect multi-scale objects effectively. In order to solve the problems of optical remote sensing image object detection mentioned above, many improved algorithms have been proposed and good detection results have been achieved. However, there is still a lack of research on object detection of large scale remote sensing images and similar objects detection between classes. At last, this paper proposes future development directions, such as improved deep learning networks, lightweight networks, weakly supervised learning, small object detection, and improved rotary detection mechanism.