下载中心
优秀审稿专家
优秀论文
相关链接
首页 > , Vol. , Issue () : -
摘要
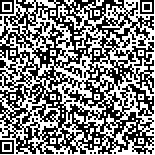
土壤侵蚀对东北黑土区的作物产量构成严重威胁,侵蚀沟是其主要表现之一。开展侵蚀沟识别和检测对东北黑土区土地综合治理等具有重要意义。本文基于多尺度稠密扩张卷积神经网络提出一个新的侵蚀沟遥感识别模型。该模型包括多个稠密连接的多尺度扩张卷积残差模块,可以更好地聚合侵蚀沟的多层级空间特征。选取黑龙江省海伦市全域作为研究区,试验结果表明本文模型取得了较好的识别效果,总体精度可达95.80%,kappa系数为0.9152,效果优于经典深度学习模型。同时,基于场景级标签和类激活图实现了对侵蚀沟区域的定位,可为侵蚀沟的边界提取提供参考。本文研究表明,基于多尺度稠密扩张卷积神经网络在东北黑土区开展侵蚀沟识别是有效的,可为东北黑土区土地综合治理提供精确的侵蚀沟空间分布数据。
Abstract: Black soil is a valuable and essential soil resource, particularly in the northeastern region of China, where it serves as the primary grain-producing area. However, the quality of local agriculture is significantly impacted by soil erosion, with erosion gullies representing a prominent manifestation of this issue. Erosion gullies, formed due to soil erosion, often interconnect within a hydrological network, creating a tree-like distribution of erosion gully systems that inflict severe damage upon cultivated land. Therefore, the accurate identification and detection of erosion gullies are pivotal for safeguarding arable land. Objective: This study explores the feasibility of utilizing remote sensing imagery for erosion gully detection and identification, leveraging its vast coverage and multiple capture instances. Additionally, we introduce a novel deep learning model tailored for erosion gully recognition based on a multi-scale dense dilated convolutional neural network. Our model incorporates dense connections of multi-scale dilated convolutional residual modules, optimized to aggregate the multi-level spatial features of erosion gullies. Method: The research was conducted within Hailun City, located in Heilongjiang Province, serving as the study area. Our approach involved cropping remote sensing images into predefined patches, which were then annotated to construct training datasets comprising two categories: erosion gullies and non-gullies. Subsequently, the model was trained on the training dataset and evaluated on the test dataset, with weight selection based on the highest test dataset accuracy. Utilizing these selected weights, we performed sliding window identification across the entire Hailun City area, thereby generating spatial distribution data for erosion gullies. Furthermore, we accomplished erosion gully area localization based on scene-level labels and class activation maps, offering guidance for boundary extraction. Results: Our findings demonstrate the efficacy of the proposed model, achieving an impressive overall accuracy of 95.80% and a kappa coefficient of 0.9152. This outperforms traditional deep learning models such as GoogLeNet, ResNet, DenseNet, and Swin-Transformer. Notably, during the sliding window recognition phase, the overall accuracy dipped slightly compared to the test phase due to the increased complexity of remote sensing imagery in practical applications. To address this challenge, we recommend incorporating a fusion of remote satellite images and street view imagery in future research to enhance recognition capabilities in complex scenarios. Conclusion: This study underscores the effectiveness of erosion gully identification through the application of a multi-scale dense dilated convolutional neural network. It serves as a valuable tool for providing precise spatial distribution data concerning erosion gullies, thereby contributing to integrated land management in the black soil region of Northeast China.