下载中心
优秀审稿专家
优秀论文
相关链接
首页 > , Vol. , Issue () : -
摘要
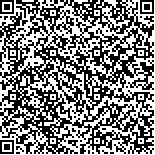
为了减轻旋转目标检测的标注负担、提高遥感旋转目标检测数据集的可用性和丰富性,本文提出了一种仅使用图像级别标注的旋转目标检测模型。该模型的核心在于利用三个一致性约束:图像级别标注的一致性、旋转检测框位置的一致性和聚类中心分布的一致性,来设计目标检测网络。首先,本文引入了遥感场景下的弱监督旋转目标检测范式,通过逐阶段优化预测结果,利用图像级别标注的一致性来训练模型。其次,为了提高旋转角度的预测准确性,模型通过利用不同旋转视图下的旋转检测框的空间位置一致性约束来调整角度预测。最后,为了保持不同旋转视图下聚类中心节点的分布一致性,设计了匈牙利损失来度量聚类中心的分布一致性。通过这些一致性约束,模型可以更好地利用不同旋转视图下的旋转等变性,提高检测性能。为了验证该模型的有效性,本文在两个主流的遥感目标检测数据集DIOR和DOTA-v1.0上进行了实验。实验结果显示,模型在DIOR数据集上取得37.7% mAP,相较于水平弱监督最先进模型提升了9.4% mAP;在DOTA数据集上取得28.1% mAP,在多个检测类别上优于难度更小的、基于水平框标注的旋转弱监督的最先进模型。这表明该模型在解决标注困难和提高遥感目标检测性能方面具有良好的潜力。未来,研究者可以进一步拓展这种基于图像级别标注的旋转目标检测模型,结合更先进的技术和不同数据源,探索其在遥感、地理空间分析和其他相关领域的更广泛应用。
Abstract: Objective: The objective of this study is to propose a novel oriented object detection model that can effectively detect objects in remote sensing images, while alleviating the challenges of labor-intensive and time-consuming annotation processes. Specifically, the aim is to develop an efficient and effective approach that only requires image-level annotations, which can improve the availability and diversity of remote sensing rotation object detection datasets. The proposed model is designed to overcome the limitations of traditional annotation methods that rely on bounding box annotations, which can be subjective, inconsistent, and time-consuming to create. By leveraging image-level annotations, the proposed model can greatly reduce the annotation effort, accelerate the annotation process, and enhance the scalability and applicability of remote sensing object detection in various scenarios and domains. Method: The proposed method introduces a novel weakly supervised oriented object detection paradigm for remote sensing scenes. The model is trained in a progressive manner, starting with coarse image-level annotations and gradually refining the detection results. This approach allows the model to learn from limited annotations and adapt to the complexities of remote sensing data, which often exhibit large scale, diverse appearance, and significant rotation variations. The model incorporates consistency constraints of image-level annotation, the oriented bounding box position and the cluster center distribution in different rotation views, to enhance the accuracy and robustness of the detection performance. The oriented bounding box position consistency ensures that the predicted rotation angles of the bounding boxes are consistent across different views of the same object, while the distribution consistency of clustering centers is measured using the Hungarian loss, which ensures that the predicted object centroids are consistent across different views. These consistency constraints are designed to improve the accuracy of the model in handling rotation variations, which is a common challenge in remote sensing object detection. Result: The proposed model is evaluated on two mainstream remote sensing object detection datasets, DIOR and DOTA-v1.0. The experimental results demonstrate that the performance of the proposed model is significantly improved compared to state-of-the-art weakly supervised remote sensing object detection models. Results show that our performance is significantly improved compared to the state-of-the-art object detectors with less strict weakly-supervised settings in remote sensing images, which also highlight the effectiveness and potential of the proposed image-level annotation-based oriented object detection model in addressing the challenges of remote sensing object detection. Furthermore, the model"s ability to generalize well to different datasets showcases its robustness and versatility. Conclusion: In conclusion, the proposed image-level annotation-based oriented object detection model is an innovative approach that addresses the challenges of labor-intensive and time-consuming annotation processes in remote sensing object detection. By leveraging image-level annotations and incorporating consistency constraints, the proposed model achieves improved detection performance while reducing the annotation effort and improving annotation efficiency. The experimental results on DIOR and DOTA-v1.0 datasets demonstrate the superior performance of the proposed model compared to state-of-the-art models, highlighting its potential for practical applications in remote sensing object detection and related fields. Future research can further explore the potential of image-level annotation-based oriented object detection models by incorporating more advanced techniques, exploring different data sources, and investigating real-world applications in remote sensing, geospatial analysis, and other related fields.