下载中心
优秀审稿专家
优秀论文
相关链接
首页 > , Vol. , Issue () : -
摘要
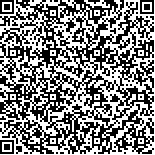
快速准确地掌握梯田的空间分布,不仅为水土保持提供重要的数据支撑,也提高了山区农业的监管水平。在利用深度学习方法进行梯田识别的过程中,形状狭长的梯田容易因卷积运算而造成漏提现象,并且在山区地物边界不清晰的复杂背景下,易产生大面积粘连的识别结果,导致最终的梯田识别精度不高。为此,本研究提出了一种基于JAM-R-CNN深度网络的梯田遥感识别方法,该网络以Mask R-CNN模型为基础,融合跳跃网络来维持高分辨率遥感影像的高语义信息,引入卷积注意力机制模块来加强梯田的特征表达能力,修改anchor size以适应梯田狭长的特性。在重庆市南川区的盐井梯田区域,使用国产高分二号(GF-2)卫星影像数据进行实验,结果表明,JAM-R-CNN网络模型的梯田识别结果精确率为90.81%,召回率为84.28%,F1为88.98%,IoU为83.15%。相较于经典的Mask R-CNN模型,JAM-R-CNN网络模型的梯田识别精度较高,4个评价指标依次提升了1.96%、5.26%、3.29%和5.19%。消融实验结果验证了模型改进的三个模块均对梯田识别有明显的促进作用。综上,本研究提出的JAM-R-CNN深度网络模型不仅能够有效减少梯田识别结果的粘连现象,而且明显提高了狭长型梯田的提取率,实现了梯田遥感识别整体精度的显著提升,具有较好的应用价值。
Efficiently and accurately grasping the spatial distribution of terraced fields not only provides important data support for soil and water conservation, but also improves the regulatory level of agriculture in mountainous areas. In the process of using deep learning methods for terrace recognition, narrow and elongated terraces are prone to missing extraction due to convolution operations, and in complex backgrounds with unclear terrain boundaries in mountainous areas, large areas of adhesive recognition results are easily generated, which leads to low accuracy in the final terrace recognition. To address the above problems, this paper proposes a JAM-R-CNN deep learning network terrace recognition method based on very high resolution remote sensing images. This network is based on the Mask R-CNN model, integrates the jumping network to maintain the high Semantic information of high resolution remote sensing images, introduces the convolutional block attention module (CBAM) to enhance the feature expression ability of terraces, and modifies the anchor size to adapt to the narrow and long characteristics of terraces, so as to achieve the purpose of improving the terrace recognition accuracy. To test the proposed method, the part of the salt well terraces in Nanchuan District, Chongqing, China was selected as the study area, and used four models in the domestic GF-2 satellite image data for experiments. These four models include the JAM-R-CNN, Mask R-CNN, Unet and DeepLab v3+ model. Finally, the precision, recall, F1 score and the Intersection over Union (IoU) were used to evaluate the accuracy of derived terraced fields. The results show that the terrace parcel map derived from the JAM-R-CNN model achieved the precision of 90.81%, recall of 84.28%, the F1 score of 88.98% and the IoU of 83.15%. Compared with the results of the Mask R-CNN, the value of the precision, recall, F1 score and IoU in the results of the JAM-R-CNN model were increased by 1.96%, 5.26%, 3.29% and 5.19%, indicating that the JAM-R-CNN model can better identify the terraces. Most of the terraces identified by the Unet and DeepLab v3+ are connected together, and the terraced fields with small size fail to be distinguished. The JAM-R-CNN model identifies fewer missing areas on the periphery of terraces compared to the Mask R-CNN model, and the number of missing narrow and long terraces is significantly reduced. This is the effect of three improvement parts, which further proves that the JAM-R-CNN model proposed in this article has significant improvement effect and shows superior performance in remote sensing recognition of terraces. In addition, some terraced fields are surrounded by commission areas, which is caused by the fact that some labels do not correctly delineate the actual boundary position of the terraced fields during the process of sample preparation. However, compared with the actual image, some of the terraced fields extracted by the experiment are more in line with the actual situation, which affects the accuracy of the final experimental results, decreasing the accuracy of the final mapping results. To sum up, the JAM-R-CNN deep learning network model proposed in this article not only effectively reduces the adhesion phenomenon of terrace recognition results, but also significantly improves the extraction rate of narrow and long terraces, achieving a significant improvement in the overall accuracy of terrace remote sensing recognition, and has good application value.