下载中心
优秀审稿专家
优秀论文
相关链接
首页 > , Vol. , Issue () : -
摘要
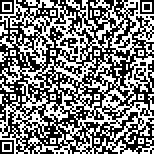
基于深度卷积神经网络(DCNN)的高分辨率遥感影像语义分割方法不断取得显著发展,但仍存在分割对象边缘特征提取与表达困难问题,导致受遮挡及小目标地物的边缘分割效果较差,从而影响语义分割方法整体精度。针对以上问题,本文提出了一种边缘感知强化的高分辨率遥感影像语义分割方法。首先,提出Transformer-DCNN协同机制提取遥感影像的全局自注意力特征和空间上下文信息,提炼更为精准的地物语义特征表达;然后,构建边缘和不确定点共同引导的边缘感知强化模块,从不确定点及实体边缘两个视角增强模型的边缘信息处理能力;最后,通过语义分割解码器有效利用包含边缘信息的特征编码,提高分割对象边缘预测的准确性和连续性。在Vaihingen和Potsdam数据集上的实验对比结果表明所提模型能有效处理复杂地物的边缘信息,提升分割方法的准确性,在常用的评估指标平均交并比(mIoU)和其他评估指标均有着不错的效果。与UNet++分割网络相比,本文所提方法在Vaihingen 数据集的mIoU得分提高了4.57%,在Potsdam 数据集的mIoU得分提高了5.01%,平均F1得分和整体精度OA也有不同程度的提升。
Objective: The semantic segmentation method of high-resolution remote sensing images based on deep convolutional neural network (DCNN) has made remarkable progress, whereas there are still problems in the extraction and expression of edge features of segmented objects. As a result, the edge segmentation effect of occluded and small target objects is poor, which affects the overall accuracy of the semantic segmentation method. Method: Aiming at the above problems, an edge-aware enhanced semantic segmentation method for high-resolution remote sensing images is proposed. First, we utilize the Transformer-DCNN collaborative feature extraction mechanism to extract the global self-attention features and spatial context information of remote sensing images, in this way, the model makes full use of the advantages of the Transformer to extract global context information and DCNN to extract spatial local context information, respectively. The proposed model extracts more accurate ground object semantic features expression, and designs a simple and effective feature extraction fusion module to fuse the features extracted by DCNN and Transformer; Next, we construct an edge-aware enhancement module that is composed of an edge-enhanced decoder and an uncertain point-enhanced decoder, which enhances the edge information processing ability of the remote sensing image semantic segmentation model from the two perspectives including uncertain points view and entity edges view; Finally, the semantic segmentation decoder effectively employs the feature codes containing edge information to improve the accuracy and completeness of segmented object edge prediction, which guarantees that the semantic segmentation effect of remote sensing images is improved overall. Results: The comparative experimental results on the two public data sets of Potsdam and Vaihingen show that compared with the original Unet++ network, the method proposed in this paper improves mIoU by 22.9%, and the average F1 score and overall accuracy also have improved in different degrees. And compared with Transformer-based TransUNet, the method proposed in this paper also achieved better results. Conclusion: The experimental results illustrated that by strengthening the feature extraction of the edge information of remote-sensing objects, the edge and overall semantic segmentation accuracy of high-resolution remote-sensing images can be effectively improved. The proposed model can effectively improve the edge segmentation accuracy of complex terrain objects, and the evaluation indicators of semantic segmentation such as average intersection ratio (mIoU) and other commonly used evaluation indicators for image semantic segmentation have good results.