下载中心
优秀审稿专家
优秀论文
相关链接
首页 > , Vol. , Issue () : -
摘要
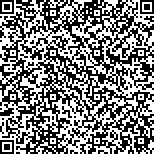
森林冠层体密度(Canopy Bulk Density,CBD)和冠层基高(Canopy Base Height,CBH)是许多火行为模型的关键输入参数。然而,在中国很少有研究关注这些参数的估算以及在区域的空间分布情况。无人机技术的发展为精细尺度估算CBD和CBH的空间分布提供了机遇。本研究首先利用野外调查数据计算样地的CBD和CBH;而后,利用无人机LiDAR点云和多光谱影像,构建基于面状区域的最优子集和随机森林估算模型,并对估算结果进行评价;最后,绘制研究区的CBD和CBH空间分布图。研究结果表明:采用相同数据源和模型时,估算CBH的R2总是高于CBD。CBD最优估算方法为融合LiDAR和多光谱数据的随机森林模型,R2为0.5142,RMSE为0.0773 kg/m3,rRMSE为40.73%。CBH的最优估算方法为仅使用LiDAR数据的随机森林模型,R2为0.6477,RMSE为1.6245 m,rRMSE为31.17%。使用单一数据源时,LiDAR估算精度明显高于多光谱数据。融合两种数据源不一定提升CBD和CBH的估算精度。本研究中构建的最优子集模型需要3-6个特征变量,随机森林模型则需要输入10-52个特征变量。仅使用多光谱影像估算CBD和CBH时,最优子集回归估算精度更好,但是空间预测结果易受地表覆盖类型的影响。本研究能够为森林冠层可燃物参数估算提供方法参考,同时也可以为林火行为预测模型提供精细尺度的输入数据。
Wildfire behavior modeling programs require spatial layers of canopy bulk density (CBD) and canopy base height (CBH) to predict fire spread. However, the both canopy fuel metrics have been investigated by few studies in China. Generally, inaccurate spatial estimates may result from the utilization of traditional field-based estimates, which assume averages across spatial extents. Recently, unmanned aerial vehicles (UAV) have emerged as valuable tools, providing LiDAR point clouds and multispectral images to estimate CBD and CBH at fine resolution. The main objective of the study is to develop an area-based approach to estimate CBD and CBH and evaluate the accuracy of various UAV datasets at a 10 m resolution at local scale in China. In this study, we set up a case study area in Jiaohe City, Jilin Province, which is predominantly covered by coniferous forests in low mountains and hills. Field data, species, crown base height, total tree height, and diameter at breast height, from 106 circular plots, which provided modeling and validation datasets. The Fire and Fuels Extension of Forest Vegetation Simulator was used to calculate CBD and CBH for each plot. The best subset regression and random forest models were employed to establish relationships between 106 field data collected and predictive variables derived from UAV LiDAR and multispectral imagery. Due to the nonlinearity of the data, we used the Box-Cox procedure to determine that a 0.5 power transformation was appropriate for the best subset regression. Our results indicate that the R2 value of CBD is always lower than that of CBH using the same models and input dataset. The fusion of LiDAR with multispectral imagery presents the best accurate estimation of CBD using random forest (R2=0.5142, RMSE=0.0773 kg/m3, rRMSE=40.73%) and LiDAR along exhibits the highest accurate estimation for CBH (R2=0.6477, RMSE=1.6245 m, rRMSE=31.17%). For both the best subset regression and random forest models, LiDAR point clouds alone had higher accuracy in estimating CBD and CBH compared to multispectral imagery. The best subset regression models exhibited greater R2 than random forest models for multispectral imagery alone. It showed that the estimated CBD and CBH values were higher using multispectral imagery than LiDAR at margin of the study area because of crop land. Fusing LiDAR with multispectral imagery uncertainly improved estimation accuracy over LiDAR and multispectral imagery alone for various models. Therefore, we recommend using the random forest of fusing LiDAR and multispectral imagery and LiDAR alone for mapping CBD and CBH in the study area due to the lowest RMSE, respectively. The best subset regression model involved 3 to 6 variables, while the random forest models encompassed 10 to 52 predictive variables. Among the original LiDAR predictor variables, height features exhibited the greatest importance, while structure features showed considerable importance. The selected multispectral imagery features of both models presented diversity for various canopy flue metrics. This study provides clear evidence that UVA LiDAR and multispectral imagery can be used to derive fine-resolution CBD and CBH, which are crucial for fire behavior modeling at a landscape scale, as well as and forest management activities and decision-making.