下载中心
优秀审稿专家
优秀论文
相关链接
首页 > , Vol. , Issue () : -
摘要
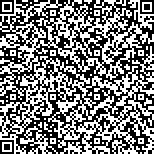
洪泛湿地的季节性变化剧烈,湿地水体和植被交替变化频繁,传统的信息提取方法存在光谱混淆和漏分的问题。本研究以Sentinel-2多时相影像为数据源,利用多尺度膨胀卷积模块改进D-UNet(Deformable U-Net)网络卷积感受野较小的缺点,以提高对高分辨率遥感影像复杂湿地结构的多尺度学习能力,基于小样本数据库训练和提取不同季节洪泛湿地的结构信息,并以新疆台特玛湖湿地为例,分析改进的D-UNet网络、5种经典语义分割模型(D-UNet、FCN8s、DABNet、Segfomer及D-LinkNet34)和传统的指数阈值法在洪泛湿地时序制图的适用性。结果表明:改进D-UNet网络在单时相影像湿地结构提取的总体精度高达96.3%,且在时序影像上具有良好的时相可迁移性和稳定性,其多时相总体精度也能达到92.3%。与其他模型及指数阈值法相比,改进后的D-UNet在洪泛湿地结构提取中表现出更好的应用潜力,对湿地水体与湿地植被的错分及漏分现象较指数阈值法分别减少了7.2%和48.9%;较改进前D-UNet分别减少了0.6%和5.4%。
Wetland is an important ecosystem and plays an important role in maintaining regional ecological security. However, the wetland structure changes respond sensitively to natural and human activities, and the flood wetlands experience drastic seasonal water and vegetation changes due to intermittent flood inundations. It is difficult to map the high-accuracy wetland structures as the frequent water and vegetation alternations lead to obvious spectral confusion and misclassification in optical satellite images. Mapping high-accuracy wetland structures is challenging due to frequent water and vegetation alternations, which cause spectral confusion and misclassification in optical satellite images. Recent studies suggest that deep learning semantic segmentation methods show great potential for mapping wetland changes in high-resolution images. In this study,a multi-scene and multi-temporal wetland sample dataset was collected using Sentinel-2 remote sensing images in the Taitam Lake wetland of Xinjiang, China. The D-UNet network was improved by replacing the convolution block before dimensionality reduction with multi-scale dilated convolutions to enhance the network"s receptive field, fuse features of different scales, and avoid loss of detailed information in high-resolution remote sensing images. The applicability of the improved D-UNet model, traditional index-thresholding methods, and four classical semantic segmentation networks for extracting wetland structural information in floodplains was compared. The results showed that the improved D-UNet network had an overall accuracy of 96.3% in single-temporal image wetland structure extraction. Moreover, it demonstrated better transferability on time-series images, with a multi-temporal overall accuracy of 92.3%. Compared to five models and the index-thresholding method, the improved D-UNet model showed better application potential in the extraction of floodplain wetland structure. It reduced misclassification and omission of wetland water bodies and vegetation by 7.2% and 48.9% compared to the index-thresholding method, and by 0.6% and 5.4% compared to D-UNet, respectively.