下载中心
优秀审稿专家
优秀论文
相关链接
首页 > , Vol. , Issue () : -
摘要
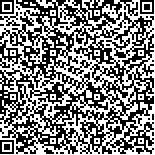
生物量是反映作物生长状况的重要指标,及时准确估计冬小麦地上生物量对于产量预测和田间管理决策具有重要意义。综合考虑遥感植被指数(Vegetation index,VI)与数字化生育期(Zadoks stage,ZS)创建的作物生物量模型(Crop biomass algorithm for wheat,CBA-Wheat)适用于全生育时期的冬小麦生物量估算,但是模型参数基于地面高光谱数据构建,而卫星遥感数据在应用过程中,需要使用更多的地面实测数据进行模型参数的调试,限制了模型的推广使用。因此,本研究采用遗传优化算法(Genetic algorithm,GA)对CBA-Wheat模型进行全局优化确定模型最优参数,利用高分辨率遥感影像提取VI与试验记录的ZS数据,构建分别以不同VI为输入变量的冬小麦生物量反演模型。结果表明:增强型植被指数(Enhanced Vegetation Index2,EVI2)为输入变量建立的模型精度最高,冬小麦生物量估算验证的决定系数(R2)和均方根误差(RMSE)分别为0.92和1.37t/ha。基于CBA-Wheat模型的生物量估算精度效果优于基于偏最小二乘回归方法的生物量估算精度(R2=0.85,RMSE=1.87t/ha)。本研究基于遗传算法优化的CBA-Wheat模型不仅具有较高的反演精度,而且适用于全生育期反演,在区域大面积生物量预测方面具有较好的应用潜力。
Biomass is an important indicator to reflect the growth status of crops. Timely and accurate estimation of aboveground biomass of winter wheat is significant for yield prediction and field management decision-making. The crop biomass model (CBA-Wheat) developed by remote sensing spectral index (VI) and digital growth stage (ZS) is suitable for the estimation of winter wheat biomass in the whole growth period. However, the model parameters are based on the ground hyperspectral data, and the satellite remote sensing data need to use more ground measured data to debug the model parameters in the application process. This limits the popularization and use of the model. Therefore, genetic algorithm is used to globally optimize the CBA-Wheat model to determine the optimal parameters of the model in this study. High-resolution remote sensing images are used to extract VI and ZS data is recorded during the field sampling. Winter wheat biomass inversion models with different VI as input variables are constructed. The results showed that the precision of the CBA-Wheat model established with the enhanced vegetation index (EVI2) as the input variable was the highest, with the determination coefficient (R2) and root mean square error (RMSE) of 0.92 and 1.37t/ha for validation results, respectively. The precision of biomass estimation based on CBA-Wheat model was better than that based on partial least squares regression method (R2=0.85, RMSE=1.87t/ha). The CBA-Wheat model optimized by genetic algorithm in this study not only has high inversion accuracy, but also is applicable to the inversion of the whole growth period, and has good application potential in the prediction of regional large area biomass.