下载中心
优秀审稿专家
优秀论文
相关链接
首页 > , Vol. , Issue () : -
摘要
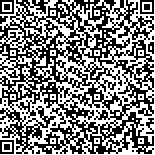
大豆是世界上最主要的作物之一,是重要的高蛋白食品、牲畜饲料的原料以及食用油来源。中国面临着大豆高度依赖于进口的粮食安全结构性挑战,对大豆的监测识别能为中国制定有效的农业经济发展策略提供基础数据支持。本研究提出了一种基于时序谱标准曲线的大豆识别方法,基于时间序列植被指数曲线并添加气象权重因子建立大豆时序谱标准曲线精确识别大豆,重点分析大豆与玉米的时序谱差异,以及气象因素对曲线的影响,并建立时序谱标准曲线信息映射到样本的方法,解决大范围大豆制图样本不足的问题。本研究设计实验验证了在时间尺度以及灾害情况下基于时序谱标准曲线识别大豆方法的鲁棒性,通过时序谱标准曲线提取的物候特征结合随机森林分类器对2020年黑龙江省大豆进行分类制图,分类混淆矩阵显示大豆识别的总体精度为86.95%,用户精度为90.91%,制图精度为86.14%,F1-Score为0.8846。本研究方法能够通过气象因子的变化表现出地域差异和灾害影响,为大豆识别(尤其是一季大豆种植区)方法适应不同研究区和灾害情况提供可行思路。
Objective Soybean is the world’s most important legume crop that serves as a major source of high-protein food, the primary ingredient for livestock feed, and an essential source of edible oil. They play a crucial role in the world"s food production, with a global annual production of around 370 million metric tons. China is one of the major soybean producing countries globally, with an annual production of around 17 million metric tons. However, China"s domestic soybean production is insufficient to meet production and living needs, and it is highly reliant on imports, accounting for more than 80% of its soybean consumption. Consequently, China"s food security faces significant structural challenges. Remote sensing technology is a powerful tool for monitoring soybean cultivation and can provide basic data support for various countries to release signals of changes in agricultural product markets, strengthen the guidance of agricultural product markets, and formulate effective agricultural economic development strategies. The traditional method of estimating soybean planting area through agricultural surveys is usually time-consuming, labor-intensive, and subject to subjective factors, leading to inaccurate and imprecise results. In contrast, remote sensing technology utilizes satellite, aerial, or drone-based sensors to capture and analyze the electromagnetic radiation reflected or emitted by the Earth"s surface, providing a more objective and efficient way of monitoring crop planting areas. While methods based on vegetation indices time series and phenology are widely used for crop recognition including soybeans, and the focus has been primarily on the impact of the vegetation index time series feature or phenological feature in soybean recognition, and there has been limited research on the time series curve itself. There is a lack of analysis and research on the spectral characteristics of the key growth stages for soybeans, and no standard spectral time series curve for soybeans has been established to summarize their changing patterns. Additionally, mainstream crop recognition methods face difficulties in obtaining samples, especially in large-scale mapping, where the quality and quantity of samples are the main limiting factors. Method This study proposes a soybean recognition method based on the standard spectral time series curve on the Google Earth Engine (GEE) cloud platform. By adding the climate weight factor, the standard spectral time series curve of soybean can be accurately recognized. Result Using the features of the standard spectral time series curve combined with the random forest classifier, a soybean distribution map in Heilongjiang Province in 2020 was extracted. The classification confusion matrix shows that the overall accuracy of soybean recognition is 86.95%, the user accuracy is 90.91%, the producer accuracy is 86.14%, and the F1-Score is 0.8846. Compared to statistical area data, the area accuracy reaches 95.94%. Conclusion The study focuses on analyzing the difference between the spectral time series curves of soybean and corn and the impact of meteorological factors on the curves, and establishes a method for mapping the standard spectral time-series curve information to samples, thereby solving the problem of insufficient samples for mapping a large area of soybean. Furthermore, this paper designs experiments to verify the robustness of this soybean recognition method based on the standard spectral time series curve in terms of time scale and disaster situations. These results provide scientific evidence and technical support for the monitoring of soybean growth, disaster evaluation, and the formulation of international agricultural product trade strategies.